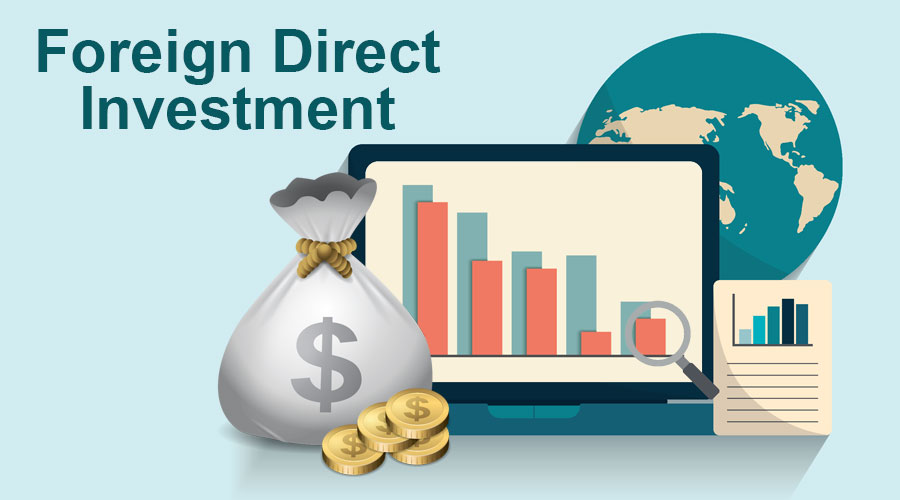
Prof. Dr., Department of Economics at Karadeniz Technical University | [email protected]; Researcher ID: AAW-8829-2020; ORCID: 0000-0001-8160-0355
2021 | vol 11(1) | 30-42
ABSTRACT
The relationship among the FDI, GDP and Export has gained vast attention among the researchers and policy-makers. There are many studies on the interaction of these variables using various econometric approaches in the literature. However, it has seen that the findings have been different from country by country. Therefore, this study’s main problematic is to estimate the coefficients that show the interaction among the FDI, GDP and Export covering 1980-2019 in Turkey. The ARDL Bounds Model and Granger Causality approach were selected to measure the coefficients statistically. Three models were executed to calculate the short-run and long-run coefficients. While the Model 1 and Model 3 were found statistically significant to explain the dependent variables, the Model 2 was found statistically insignificant. Because of this, the Model 2 was excluded from the study. The short-run coefficients were also found statistically significant to explain the dependent variables of the Model 1 and Model 3. While GDP affects the FDI positively in Model 1, GDP affects the Export negatively in Model 2. The ECT was found statistically significant at 0.01. The speeds of adjustment of the Model 1 and Model 3 were calculated as approximately 93% and 16% levels, respectively. Unlike the ARDL Bounds Model, the Granger Causality test was implemented to measure the variables’ causal relationship. It was seen that there is only a unidirectional Granger causal relationship running from GDP to FDI in the Model 1 and from GDP to Export in the Model 2.
Keywords: Autoregressive Distributed Lag Bounds Model (ARDL), Granger Causality, Export, FDI, GDP
Introduction
Foreign Direct Investment (FDI), Gross Domestic Product (GDP) and Export, to some extent, affect the economic growth and development such as investment, physical equipment, technology, human capital, knowledge spillovers. As it is known that the Less Developed Countries are in the permanent lack of convertible external saving which can be used as a payment tool in international trade. Even if domestic saving is sufficient in the internal market, they need to have convertible external saving to import the items used in their production process. In order to eliminate this problem, if the lending, grant and humanitarian aid are ignored, the most preferred options of the countries are attracting the FDI into the domestic economy and increasing export revenues from the international trade.
In the classical growth model, the capital should move from the developed countries to the less developed countries because of the diminishing returns of capital. However, FDI goes largely to the developed countries and markets with high GDP. This situation is called as “Lucas Puzzle” in economic theory (Lucas, 1990). In the neoclassical growth model (Solow, 1956); (Romer, 1988), economic growth is broadly based on capital accumulation and total factor productivity. In this context, FDI can be seen as a substantial source of capital accumulation which complements the growth efforts in the country.
Domestic companies have much more information and access opportunities for the domestic market. If a foreign firm decides to enter the domestic market, it has to compensate for the advantages of domestic companies. For this reason, foreign companies have to get lower cost and high efficiency to eliminate this unwanted situation. Because of this, The FDI is generally accepted as more efficient than domestic investments. Nevertheless, it is difficult to attract FDI into the domestic economy. There are many factors affecting FDI inflows such as infrastructure, the regime type, the rule of law, political stability, the quality of labour force, effective tax and wage rates, etc. The market size is accepted as the most determining factor when comparing to the others. The variable representing the market size is the GDP level. The GDP affects both FDI inflows and Export simultaneously.
It should not be forgotten that the most reasonable form to eliminate the external saving gap is to get export revenues from foreign trade transactions. Moreover, the export revenues depend on the productivity and efficiency levels of the economy. If the policies on FDI are formed as the necessities of the host economy, FDI can affect the competition power of the host economy via increasing productivity and efficiency levels. So, the study’s main problematic is to estimate the coefficients that show the interaction among FDI, GDP and Export by using Autoregressive Distributed Lag (ARDL) Bounds Model and Granger Causality test for Turkey covering 1980-2019.
- Factsheets
It can be stated that FDI is one of the most crucial instruments to strengthen the competitive power of the host countries by improving the efficiency and productivity of the domestic economy against the international economic powers.
The FDI inflows into Turkey over the period of 1990-2000 remained stable at a low level. After the 2000 Crisis, The FDI inflows into Turkey have increased gradually. Applied economic policies transform the Turkish Economy more open to foreign competition in this period. It is possible to say that FDI inflows reached the highest level in the period of 2006-2008. Although encouraging economic policies have been implemented, it can be stated that Turkey has failed to attract the FDI sufficiently. Essentially, Turkey is actually quite a good position in attracting FDI into the domestic market. However, when considering Turkey’s economic potential and geostrategic position, it can not be said that Turkey is very successful for attracting FDI. In this process, it is necessary to say that Turkey has been subjected to the political obstacles unrelated to the economic truths as well. The FDI Inflows into Turkey is displayed in a figure as follows:
FDI Inflows (1980-2019, US$)
There is approximately $1.5 trillion of FDI circulated throughout the World in 2019. More than half of FDI (51.9%) went to the developed countries and markets with high GDP level. It is seen the same tendency in country data as well (USA-$246 billion, China-$141 billion, Singapore-$92 billion, Netherlands-$84 billion, United Kingdom-$64 billion). Turkey attracted approximately $8.4 billion of FDI into the domestic market at the same year, equal to 0.5% in total. FDI inflows by groups of economies in 2019 are as follows:
FDI Inflows by Groups of Economies, 2019
Group of Economies | FDI Inflows
(millions, $) |
(%) | |
Developed Countries | 800.239 | 51,9 | |
Developing Countries | 684.723 | 44,5 | |
Less Developed Countries | 21.147 | 1,4 | |
Others | 33.771 | 2,2 | |
The World | 1.539.880 | 100 | |
UNCTAD World Investment Report 2020, page: 8
As seen from the table that if a country is developed, it attracts more FDI into the domestic economy. Therefore, it can be said that one of the most critical indicators affecting the FDI movements is the market size, which is measured in terms of GDP. The structurally weak, vulnerable and small economies with limited market size have been subjected to insufficient FDI inflows to eliminate the saving gaps.
According to the World Bank and IMF data, Turkey’s GDP in 2019 is worth 754.412 billion dollars, which corresponds to the 0.63 per cent of the world economy. While Turkey wathe 17th largest economy in the World in GDP at current prices in 2019, it is the 13th largest economy regarding the GDP, calculated by the Purchasing Power Parity (PPP). GDP (PPP) in Turkey is 2.325,617 million of international dollars in 2019. Besides this, Turkey is the 6th largest economy in rankings of the same year compared to the EU countries. As seen from Figure A, the Turkish economy has remarkably performed well since the 2000 Economic Crises. The average growth rate of this period is approximately 5 per cent level. The visible improvements in the Turkish Economy have also triggered foreign trade positively. Figure B shows the export performance of Turkey. The export performance of Turkey is approximately worth 180 billion dollars in 2019. 31.6% of GDP was exported to the foreign markets in the same year. According to the World Bank’s official data, Turkey is the 26th in the rankings of World export in 2019. GDP (constant, 2010, US$) and Export (constant, 2010, US$) in Turkey are shown in figures as follows:
Figure A) GDP (constant, US$) Figure B) Export (Constant, US$)
- Literature
There has been a spate of research in literature deriving the econometric relationship among FDI, GDP and Export. The various methods have been used so as to estimate these interactions. According to the results of the selected studies, it can be stated that the results of the studies are inconclusive.
Afzal and Khan (2017) used the ARDL Bounds Model to investigate the impacts of Export on Economic Growth for Pakistan over the period of 1972-2014. In this study, Export, Import, CPI and Terms of Trade were selected as independent variables to explain the dependent variable, GDP. The results showed that while Import, CPI and Terms of Trade negatively affect economic growth, Export has a positive impact on Economic Growth. Bellouni (2014) used the ARDL Bounds Model, Granger Causality and Cusum test to estimate the dynamic relationships between Labor, Trade Openness, FDI, Capital Investment and Economic Growth in Tunisia covering 1970-2008. The results show that Trade Openness and Economic Growth promote FDI in the long-run. There is no significant Granger causal effect from FDI to Economic Growth and vice versa. In addition to this, there is no Granger causal effect from Trade Openness to Economic Growth in the short-run and vice versa. Modou and Liu (2017) examined the interactions between FDI, Trade Openness and Economic Growth in 13 West African Countries from 1980 to 2015. They used Fully Modified Ordinary Least Squares (FMOLS) method to estimate the relationships. The outcomes of the model show that Trade Openness and FDI affect Economic Growth positively.
Kalai and Zghidi (2017) analyzed the relationship between FDI, International Trade, and Economic Growth using ARDL Bounds Model and VECM approach for the 15 selected Middle Eastern and North African Countries (MENA) covering 1999-2012. The findings show that there is a long-run unidirectional relationship from FDI to Economic Growth in MENA countries. In addition to this, there is also found that the FDI can generate positive spillover externalities for these countries. Aqeel and Nishat (2014) identified the determinants of FDI growth in Pakistan for the period 1961-2003. Tariff Rate, Exchange Rate, Tax Rate, Credit to Private Sector and Index of General Share Price variables were used to the estimation. The Ordinary Least Squares, Cointegration and Error Correction Technique (ECT) were used for the estimation. The variables affect both FDI inflows and Economic Growth positively in both short-run and long-run in Pakistan. Borensztein et al. (1998) tested the effect of FDI on Economic Growth using a cross-country Seemingly Unrelated Regression-SUR for 69 developing countries covering 1970-1979 and 1980-1989. The study suggests that FDI is an important vehicle to transfer the technology from abroad to the internal economy. Bouchoucha and Ali (2019) attempted to examine the impacts of FDI on Economic Growth in Tunisia using ARDL Bounds Model for the period from 1980 to 2015. The findings show that FDI has a positive impact on Economic Growth in Tunisia in both the short-run and long-run. Domestic Investment and Human Capital also have positive effects on Economic Growth in the same period. The degree of Trade Openness has a negative effect on Economic Growth in both the short-run and long-run. Zubaudi et al. (2007) investigated the role of FDI on Economic Growth in Malaysia by using ARDL Bounds Model covering 1970-2004. The findings show that Domestic Capital Formation, FDI, Human Capital, and Financial Deepening positively affect the Economic Growth. Carbonnel and Werner (2018) analyzed the link between Economic Growth and FDI by using ARDL Bounds Model, Pairwise Granger Causality, Two-stage OLS and GETS (General to Specific) tests covering 1984-2010 for Spain. FDI, Credit, Bank Lending, International Commodities Index, Total GDP of G7, EUR/DM Exchange Rate, EUR/USD Exchange Rate, Employed Spanish Population, Educational Level of Employed Population, M1, M2 and Spanish Overnight Interest Rates were determined as the variables of the study. It was found that there is no causal relationship from FDI to Economic Growth.
Goah and Sam (2017) examined the long-run relationship among the FDI, Export and GDP in selected Asian economies using the bootstrap ARDL Bounds Model and Granger Causality tests. The tests failed to find the existence of cointegration when GDP is taken as a dependent variable. According to the findings, the FDI and Export are not the sole sources of economic growth in India, Indonesia, Japan, Korea, Malaysia, Philippines, Singapore, Taiwan, Thailand, China and Hong Kong. It was also found from the Granger Causality test that GDP affects the FDI inflows positively. Canal-Fernandez (2018) examined the relationship between FDI, Export and Economic Growth in Spain by using ARDL Bounds Model for 1970-2016. The ECM and VECM were determined as the estimation methods. The results show that there is a long-run relationship between the variables. There is a strong unidirectional causal relation from FDI to Export. In addition to this, there is no causal relationship from FDI to Economic Growth and vice versa. Dritsaki and Stiakakis (2014) investigated the relationship between Export, FDI and Economic Growth in Croatia using annual time series data for 1994-2012. The ARDL Bounds Model and ECM-ARDL model were determined as the estimation. The results show that there is a bidirectional short-run and long-run relationship between Export and Economic Growth. Anshul (2013) examined the dynamic causal relationship among Economic Growth, FDI, Trade and Capital Stocks covering 1970-2012 using the ARDL Bounds Model, Granger Causality and Cusum test for India. The findings show that the Capital Investment and FDI promote the Economic Growth in the long-run, and there is no Granger causal effect from FDI to Capital Investment and vice versa. Etale and Etale (2016) examined the relationship between Export, FDI and Economic Growth using Johansen cointegration and VECM tests covering 1980-2013 in Malaysia. The findings show that there is a significant bidirectional relationship between FDI inflows and GDP. Sultanuzzaman et al. (2018) examined the short-run and long-run relationship among FDI inflows, Export and Economic Growth using ARDL Bounds Model in Sri Lanka covering 1980-2016. The study showed that FDI inflows affect Economic Growth positively in both the short-run and long-run. However, Export has a negative effect on economic growth in the short-run and long-run. If Export increase, The GDP growth will decrease. Khan (2020) analyzed the relationship between FDI inflows and Economic Growth using the ARDL Bounds Model and Granger causality tests covering 1972-2017 in Bangladesh. The ARDL Bounds Model reached a long-run relationship between variables. Besides this, there is a unidirectional Granger causal relationship from GDP to FDI. Klasna (2019) examined the relationship between FDI, Trade Openness and Economic Growth using the ARDL Bounds Model covering 1975-2004 in Pakistan and Turkey.
The results indicated that there is a bidirectional relationship between Trade Openness and Export for Pakistan. Moreover, there is also found a bidirectional relationship between FDI and Export for Turkey. Shimul et al. (2009) examined the relationship between FDI and GDP in Bangladesh by using the ARDL Bounds Model and Engle-Granger two-step tests over the period 1973-2007. The results show that there is no cointegration between the variables. The FDI and Openness have no causal effect on GDP. Shahid and Pentecost (2007) examined the determinants of FDI inflows using the ARDL Bounds Model the period 1973-2004 for Pakistan. The results show that GDP is the main determinant to attract FDI into the domestic economy in the long-run. The political risk is the other factor affecting FDI inflows in the short-run for Pakistan as well. Gudaro et al. (2012) analyzed the relationship among GDP, FDI, and CPI using the Multiple Regression Model covering 1981-2010 in Pakistan. According to the findings of the model, FDI has a positive effect on GDP. Uwubanmiran and Ogiemudia (2016) examined the effects of FDI on Economic Growth by using ECM in both short-run and long-run in Nigeria. The time-series data cover the period 1979-2013. The study revealed that FDI affects the economy in the short-run and has a non-significant negative effect in the long-run. According to the Granger Causality test, FDI has a significant positive effect on Economic Growth in Nigeria. Nguyen (2017) examined the long-run effect of FDI and Export on the Economic Growth in Vietnam for 1986-2015. The ARDL Bounds Model and ECM were implemented to the estimation. The study shows that FDI is an important factor affecting Economic Growth positively, while Export negatively affects economic growth.
- Data and Methodology
3.1. Data
This paper used annual time series data on FDI (net inflows, BoP, constant, 2010, US$), GDP (constant, 2010, US$) and Export (constant, 2010 US$) covering the period of 1990-2018. FDI and Export variables were deflated by the GDP deflator, which was constructed specifically for this prediction. The variables were also expressed in natural logarithm levels as LNFDI, LNGDP and LNX. The data were taken from the World Development Indicators (WDI) released from the World Bank. In this paper, the ARDL Bounds Model and Granger Causality approach were used for the estimation.
3.2. Methodology
- Unit Root Test
Many studies on time series require the stationarity tests to transform the data to be stable. If a time series is stationary, the mean, variance and covariance of the series do not vary in the time process. Essentially, there are several tests used to determine the stationary in practice. In this paper, the stationary was measured by the applying Augmented Dickey-Fuller (ADF) and Phillips-Perron (PP) tests. In these tests, if the critical value is lower than the estimated value, the null hypothesis is rejected, and the series is accepted as stationary.
Table 1
Unit Root Tests
Intercept | Trend and Intercept | ||||||||||||
ADF | ADF | PP | PP | ADF | ADF | PP | PP | ||||||
(Level) | (1st Dif.) | (Level) | (1st Dif.) | (Level) | (1st Dif.) | (Level) | (1st Dif.)
|
||||||
-2.4774
(0,1286) |
-8.0229***
(0.0000) |
-2.6425
(0.0934) |
-12.1251***
(0,0000) |
-3.9203***
(0.0205) |
-18.9165
(0.0000) |
-3.9529***
(0.0189) |
-18.9165
(0.0000)
|
||||||
-1.0695
(0.7181) |
-5.5982***
(0.0000) |
-1.1182
(0.6990) |
-5.6069***
(0.0000) |
-1.2227
(0.8917) |
-5.5988***
(0.0003) |
-1.6580
(0.7507) |
-5.5914***
(0.0003)
|
||||||
-2.6194
(0.0977) |
-5.8287***
(0.0000) |
-2.5778
(0.1061) |
-6.2131***
(0.0000) |
-5.6704***
(0.0002) |
-5.7309
(0.0002) |
-5.7309***
(0.0002) |
-6.0147
(0.0001)
|
||||||
(***); stationary of the variable at 0.01 level; values in brackets are the probability values of the variables.
As seen from Table 1, in the “Model with Intercept”, FDI, GDP and Export were found stationary at 1st difference at 0.01 in both ADF and PP tests. In the “Model with Trend and Intercept”, while FDI and Export were found stationary at level in ADF, GDP was found stationary at 1st difference. In PP test, the results are similar to the ADF test. While FDI and Export were found stationary at level, GDP was found stationary at 1st difference. Schwarz Information Criteria (SIC) was used to determine the optimal lag orders for all variables as well.
- ARDL Bounds Model
The ARDL Bounds Model is developed by Peseran et al. (2001). The model examines the short-run and long-run effects of variables empirically. It is accepted that the ARDL Bounds Model possesses some advantages comparing to the other cointegration tests such as Engle-Granger, Johansen and Johansen-Juselius. Working more efficiently in the small size of data, unbiased estimate in the long-run, and not requiring the same order integration of variables have been accepted the chief advantages of this model.
The ARDL Bounds Model involves two steps. The first step is the estimation of the existence of the long-run relationship, and the second step is the estimation of the short-run relationship by using error correction regression.
Hypotheses of the models are shown as follows:
(Cointegration does not exist.)
(Cointegration exists.)
Table 2
The Results of the ARDL Bounds Model
Model | f-bounds test
( Value) |
f- statistics
( Significant) |
finite sample: n=40
I(0) I(1) |
|
Model 1 | 9.360277 | 10%
5% 1% |
3.373
4.133 5.893 |
4.377
5.26 7.337
|
Model 2 | 1.946795
|
10%
5% 1% |
3.373
4.133 5.893 |
4.377
5.26 7.337
|
Model 3 | 4.762506 | 10%
5% 1% |
3.373
4.133 5.893 |
4.377
5.26 7.337 |
There were three models created in the ARDL Bounds Model. The models can be expressed as follows:
The ARDL Bounds Model unveils long-run associations between variables. In the guideline, if the f-value exceeds the critical value’s upper bounds, there is cointegration between variables in the long-run. It means that all variable move together in the long-run as well. If the f-value is between upper and lower bounds levels, it can not be said something to explain the long-run associations. This interval is called as the area of uncertainty. If the f-value is under the lower bound levels, there is no relationship between variables in the long-run.
The f-value of Model 1 was measured as 9.360277. It is higher than the upper bounds values at 0.01, 0.05 and 0.10 levels. It means that there is a long-run associations between variables which are FDI, GDP and Export. They are all cointegrated and move together in the long-run. The f-value of Model 2 was measured as 1.946795. It is smaller than the upper bounds of the model at 0.01, 0.05 and 0.10 levels. It means that there is no long-run associations between variables. The variables are not cointegrated in the long-run. They do not move together as well. The f-value of Model 3 was measured as 4.762506. It is higher than upper bounds levels only at 0.10 (4.762506 > 4.377). It means that the model is significant at 0.10. There is a long-run associations between the variables at this level. All variables are cointegrated and move together in the long-run.
Table 3
The Long-run Coefficients of the Models
Model | Variable | Coefficient | Std. Error | t-statistics | Prob. |
D(LNFDI) | LNGDP | 0.459066 | 0.620558 | 0.739764 | 0.4656 |
LNX | 1.508139 | 0.431773 | 3.492894 | 0.0016(***)
|
|
D(LNGDP) | LNFDI | 0.377709 | 0.244067 | 1.547765 | 0.1310 |
LNX | -0.476949 | 0.590862 | -0.807208 | 0.4252
|
|
D(LNX) | LNGDP | 0.004950 | 0.553277 | 0.008946 | 0.9929 |
LNFDI | 0.317256 | 0.183951 | 1.724680 | 0.0937(*) |
(***), (**), (*); significance at 0.01, 0.05 and 0.10 levels, respectively
The coefficient of GDP was found as statistically insignificant to explain the dependent variable of FDI in Model 1. It means that there is no long-run relationship between GDP and FDI statistically. The coefficient of Export of the Model 1 was found as positive and statistically significant at 0.10. It means that there is a long-run relationship between Export and FDI. The GDP coefficient of Model 3 was found statistically insignificant, which means that there is no long-run relationship between GDP and Export. FDI coefficient of the Model 3 is statistically significant at 0.10. It shows the long-run relationship between FDI and GDP. The Model 2 had been excluded from the paper, because of the f-value of the model was found lower than the upper bounds values.
- Error Correction Model
The ECM is used to eliminate the imbalance between the short-run and long-run relationships in time series analysis and test the short-run and long-run causalities between cointegrated variables. The models are shown as follows:
Table 4
The Short-run Coefficients of the Models (Unrestricted Constant and No Trend)
Model | Variable | Coefficient | Std. Error | t-stat. | Prob. |
Model 1
(FDI) |
C |
-11.67316(***) |
2.147194 |
-5.436470 |
0.0000 |
D(LNGDP) | -0.253723 | 0.494236 | -0.513365 | 0.6117 | |
D(LNGDP(1)) | 0.650340 | 0.470677 | 1.381710 | 0.1780 | |
D(LNGDP(2)) | 0.826763 | 0.480371 | 1.721093 | 0.0963 | |
D(LNGDP(3)) | 1.828285(***) | 0.498237 | 3.669508 | 0.0010 | |
CointEq(-1) | -0.644308(***) | 0.117481 | -5.485126 | 0.0000 | |
Model 3
(EXPORT) |
|||||
C | 3.014855(***) | 0.748896 | 4.025732 | 0.0003 | |
D(LNGDP) | -0.652159(***) | -1.32258 | -4.930968 | 0.0000 | |
CointEq(-1) | -0.159281(***) | 0.040952 | -3.889468 | 0.0004 |
(***); significant at 0.01 level
The short-run coefficients of the Model 1 and Model 3 were found statistically significant to explain the dependent variables. While it was found a positive relationship between GDP and FDI, it was found a negative relationship between GDP and Export. In the guideline, the coefficient of ECT should be negative and statistically significant. As seen in Table 4, The Error Correction terms of the Model 1 and Model 3 are compatible with the guideline. The Error Correction terms were found both negative as -0.644308 and -0.159281, respectively. The coefficients indicate the speeds of adjustment of the models. According to the findings, the systems of the Model 1 and Model 3 were adjusted towards the long-run equilibrium approximately at the speeds of 64% and 16% in the corresponding period, respectively. The results also unveil that the Error Correction coefficients show the existence of a stable long-run relationship between the variables. So, all the variables are cointegrated and move together in the long-run.
- The Cusum and the Cusum Square Tests
It is accepted that the Cusum and the Cusum Square tests, which can be calculated by the consecutive errors are valid and reliable tests for evaluating the stability of the long-run relationships between the variables. It gives us rough information about whether or not there are breaks in the data set. If there is a break in data set, it can be fixed using the Cusum Square test.
The graphs show the stability of coefficients of the models for the period of 1990-2018. As seen from the graphs, there is no structural break in the long-run. Therefore, it is accepted that both the Model 1 and Model 3 are stable concerning the Cusum and the Cusum Square tests displayed as follows respectively:
Model 1
The Cusum and the Cusum Square Tests
Model 3
The Cusum and the Cusum Square Tests
- Granger Causality Test
The study applied to the Granger Causality test to ascertain the causal relationship between variables. The Granger Causality test is a statistical method for investigating information between time series. If the p-values are higher than 0.05, the null hypothesis can not be rejected, which means that there is no Granger causal relationship between variables and vice versa. If the p-value is less than 0.05, the null hypothesis can be rejected, which means that there is a Granger causal relationship between variables and vice versa. The results of the Granger Causality test are as follows:
Table 5
The Results of VAR Granger Causality/Block Exogeneity Wald Tests
Model | Chi-Sq. | Prob. |
LNFDI
LNGDP |
10.95866(***) |
0.0009 |
LNX | 3.212822 | 0.0731 |
All
LNGDP
|
14.20860(***) | 0.0008 |
LNFDI | 2.137327 | 0.1438 |
LNX | 5.95E-05 | 0.9938 |
All
LNX
|
5.398296 | 0.0673(*) |
LNFDI | 2.099993 | 0.1473 |
LNGDP | 7.289292(***) | 0.0069 |
All | 7.291810 | 0.0261(**) |
(***), (**), (*); significance at 0.01, 0.05, and 0.10 respectively
|
According to the Granger Causality Test, the p-value of the GDP coefficient in Model 1 was 0.0009, which is less than 0.05. The lag order was found as 1. It is statistically significant to explain the dependent variable, which is FDI. It means that there is a Granger causal relationship running from GDP to FDI. The p-value of the Model 3 was found as 0.0069, which is less than 0.05. It means that the coefficient of the GDP is statistically significant to explain the dependent variable, which is Export.
Conclusion
This paper investigated the FDI, GDP and Export nexus in the Turkish Economy covering 1980-2019. The ARDL Bounds Model and Granger Causality test were used to estimate the short-run and long-run relationship between FDI, GDP and Export. Three models were constructed in order to reach the relationship between the variables in both short-run and long-run. According to the findings, the f-value of Model 1 was 9.360277, which is greater than the upper bounds values of the test at all levels. It means all variables are cointegrated and move together in the long-run. Model 2 was dropped from the paper. Because the model was found statistically insignificant, thanks to the fact that the f-value of the model is less than the upper bounds values for all levels. The f-value of the Model 3 was found as 4.762506 and statistically significant only at 0.10.
The long-run coefficients of the models were estimated. According to the findings of Model 1, the probability value of Export coefficient was found as 0.0016. It is statistically significant at 0.01. GDP is not statistically significant to explain the dependent variable. The probability of the coefficient of GDP was found as 0.4656. It is higher than 0.05. As for the Model 3, the long-run coefficient of GDP was found as 0.004950. The probability value of the coefficient is 0.9929. It is higher than 0.05. Therefore, the GDP variable was found statistically insignificant to explain the Export. The coefficient of FDI was found as 0.317256. The probability value of this is 0.0937. So, FDI was found statistically significant to explain Export in the long-run at 0.10.
The short-run coefficients were statistically significant in explaining the dependent variables of the Model 1 and Model 3. According to the result of the Model 1, it was found that the GDP affected FDI positively. So, if the GDP increases, the FDI inflows also will increase. Besides this, it was also found a negative relationship between the GDP and the Export in Model 3. If the GDP increases, Export will decrease. It was estimated the Error Correction coefficients of the models. The coefficients of the Model 1 and Model 3 were found as -0.932295 and -0.159281, respectively. The signs of the coefficients are negative and statistically significant at 0.01. According to the guideline, ECT should be negative and statistically significant. In the model, the systems were adjusted towards the long-run equilibrium approximately at the speeds of 93% and 16% respectively.
The Cusum and the Cusum Square tests were carried out to get both models’ structural breaks. The findings show that the models are stable, in which there is no structural problem in both models. According to the VAR Granger, Causality/Block Exogeneity Wald test indicated that there are some meaningful unidirectional Granger causal relationships from GDP to FDI and from GDP to Export in Turkey throughout1980-2019.
References
Ahmad D, Afzal M and Khan UG (April 2017), Impact of Export on Economic Growth: Empirical Evidence of Pakistan, International Journal of Applied Economic Studies.Vol.5, Issue.2.
Akeel A and Nishat M (2014), Determinants of Foreign Direct Investments in Pakistan: Is China Crowding Out FDI Inflows in Pakistan. Pakistan Development Review. 43(4), pp: 651-664.
Anshul S (2013), Do the Foreign Direct Investment, Economic Growth and Trade Affect Each other for India: An ARDL Approach, MPRA-Munich Personal Repec Archive, Online at https://mpra.ub.uni-muenchen.de/51447/
Bellouni M (2014), The relationship between Trade, FDI and Economic Growth in Tunisia: An Application of the Autoregressive Distributed Lag Model. Economic Systems. 38 (2014), pp: 269-287.
Bibuhti S and Khan F (2020), Nexus between FDI and Economic Growth in Bangladesh: an augment autoregressive distributed lag Bounds Model approach, Financial Innovation Vol (6), pp: 1-18.
Borensztein E, De Gregorio J and Lee JW (1998), How Does Foreign Direct Investment Affect Economic Growth. Journal of International Economics. 45 (1998), pp: 115-135.
Bouchoucha N and Ali W (2019), The Impact of FDI on Economic Growth in Tunisia: An Estimate by the ARDL Approach. MPRA Papers No.91465.
Buharumshah AZ and Almasuided SW (2009), Foreign Direct Investment and Economic Growth in Malaysia: Interactions with Human Capital and Financial Deepening. Emerging Market Finance and Trade. Vol.45, No.1, pp: 90-102.
Canal-Fernandez V (2018), The long-run impact of Foreign Direct Investment, Export and GDP: Evidence from Spain from an ARDL Approach, EHES Working Paper Series No. 128.
Carbonel, JB and Werner RA (2018), Does FDI Generate Economic Growth? A New Empirical Approach Applied to Spain. Economic Geography.Vol.94, Issue.4.
Etale E and Etale LM (2016), The Relationship between Export, Foreign Direct Investment and Economic Growth in Malaysia, International Journal of Business Management and Economic Research, Vol. 7 (2), pp: 572-578.
Goah, S K and Sam CY (2017), Re-examining Foreign Direct Investment Export and Economic Growth in Asian Economics using Bootstrap ARDL Test for Cointegration. Journal of Asian Economics. Vol 51(c), pp: 12-22.
Gudaro AM, Chhapro IU and Sheikh, SA (2012), Impact of Foreign Direct Investment on Economic Growth: A Case Study of Pakistan, Journal of Management and Social Sciences, Vol.8, No. 2 (fall 2012), pp: 22-30.
Kalai M and Zghidi N (2017), Foreign Direct Investment, Trade and Economic Growth in MENA Countries: Empirical Analysis using ARDL Bounds Model Approach, Journal of the Knowledge Economy 10(1), pp: 397-421.
Khan, BSF (2020), Nexus between Foreign Direct Investment and Economic Growth in Bangladesh: an augmented autoregressive distributed lag bound testing approach. Financial Innovation, 6 (10).
Klasna MA (2011), Foreign Direct Investment, Trade, Openness and Economic Growth in Pakistan and Turkey: An Investigation using Bound Test. Qual Quant. 45:223-231.
Lucas RE (1990), Why Doesn’t Capital Flow from Rich to Poor Countries? The American Economic Review, 80 (2), pp: 92-96.
Mahmood Shah IU and Khan FU (2014), Domestic Investment, Foreign Direct Investment and Economic Growth Nexus: A Case of Pakistan. Economic Research International. 2014 (1), pp: 1-5.
Modou D and Liu HY (2017), The Impact of Asian Foreign Direct Investment, Trade on Africa’s Economic Growth, International Journal of Innovation and Economic Development, Vol.3, Issue.1, pp: 72-85.
NGuyen NTK (2017), The Long-run and Short-run Impacts of Foreign Direct Investment and Export on Economic Growth of Vietnam. Asian Economic Financial Review. Vol.7, No.5, pp: 519-527.
Peseran MH, Shin Y and Smith RJ (2001), Bounds Model Approaches to the Analysis of Level Relationships. Journal of Applied Econometrics. 16 (3), pp: 289-326.
Raza SS and Hussain A (Summer 2016), The Nexus of Foreign Direct Investment, Economic Growth and Environment in Pakistan. The Pakistan Development Review. 55:2, pp: 95-111.
Romer PM (1988), Capital Accumulation in Theory of Long-run Growth. RCER Working Papers 123, the University of Rochester-Center for Economic Research.
Shahid HM and Pentecost E (2007), A Time Series Econometric Model of Determinants of The Level of Inward Foreign Direct Investment into Pakistan. Indian Economic Review. Vol.XXXXII, No.2, pp: 177-190.
Shimul SN, Abdullah SM and Siddiqua S (2009), An Examination of FDI and Growth Nexus in Bangladesh: Engle-Granger and Baund Testing Cointegration Approach. Brac University Journal. Vol. VI, No.1, pp: 69-76.
Solow RE (1956), A Contribution to the Theory of Economic Growth. The Quarterly Journal of Economics. Vol.70, Issue 1, pp: 65-94.
Sultanuzzaman M, Fan H, Wang B and Shakij US (2018), The Role of FDI Inflows and Export on Economic Growth in Sri Lanka: An ARDL Approach. Cogent, Economic and Finance. Vol. 6 (1), pp: 116-151.
Uwubanmuran AE and Ogiemudia OA (2016), Foreign Direct Investments and Economic Growth: Evidence from Nigeria. International Journal of Business and Social Science, Vol. 7, No.3, March 2016, pp: 89-103.