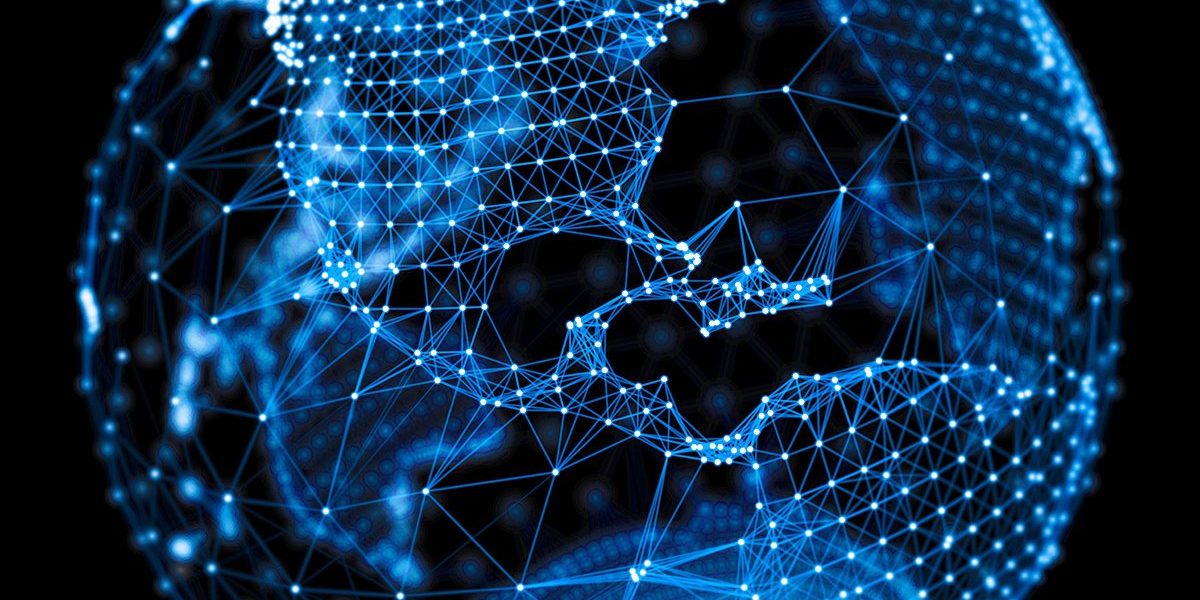
RESEARCH ARTICLE the rest volume 9, number 2, summer 2019 | ||
The Role of Social and Economic Factors on Fast Food Restaurant Chains M. Yunus Sisman* & Hakan Uslu** * Assist. Prof. at the Department of Economics, Dumlupinar University. ** Assist. Prof. at the Department of Economics, Adiyaman University. |
||
ARTICLE INFO | ABSTRACT | |
Keywords: Count data models Received 20 December 2018 |
This paper explores how supply of the fast food restaurants is distributed in the US metropolitan areas by using spatial and count data models at the census-tract level. We empirically investigate the relationship between the number of fast food restaurants and socioeconomic status (i.e., race, gender, income inequality, educational attainment, and unemployment) in US cities. Findings suggest that income inequality stimulates the prevalence and the density of the fast food restaurants. Among the other neighbourhood and demographic characteristics, low income and black population dominated census-tracts are more attractive for fast food chains. Another key finding is the number of the fast food restaurants increases around the public and private schools in the census tracts. |
Introduction
Every day, 1 in 4 Americans visits a fast food restaurant. More than 50,000,000 people in U.S. depend on fast food (www.ffood.net). The percentage of calories gained from fast-food consumption has increased from 3% to 12% in the last 20 years (Lin and Frazao, 1999). Hence, Americans are now the fattest people on the earth and 6 out of every 10 of them (a quarter of their children) are now overweight (Critser 2003). Over the last thirty years, fast-food retail sales in the U.S. have risen continuously from $16.1 billion in 1975 to $169.8 billion in 2012 (Technomic 2004). The number of fast-food restaurants in the country reached to 263.944. Studies report that Americans are spending more on fast food than on new cars, computers, computer software or even higher education (Schlosser 2002).
This study aims to outline the link between the fast-food restaurant density and economic and social characteristics in the US metropolitan areas struggling with obesity at the most disaggregated level (i.e., census-tract level). In particular, we study Montgomery, AL which is the most obese city in the US (Gallup and Healthway Survey, 2009). A central question that we address is whether the demographic and socioeconomic characteristics of a city affect the supply of fast food chains. The question is extended to analyze the effects of school locations on prevelance and density of fast food restaurants. Therefore, our research includes a geographical analysis to combine demographic, economic, and geographical data for each census tract in the cities. In addition, this analysis allows us to obtain data for locational interrelations between fast food restaurants and school zones. Then, we employ an econometric estimation to explain the impacts of neighbourhood characteristics on fast food supply.
The empirical findings of this study suggest that among others, the ethnicity and income inequality are the most important factors on prevelance and density of fast food restaurants. Results show that the fast-food restaurant density is higher in neighbourhoods with predominantly black population and higher income inequality. Results further indicate that the number of the fast food restaurants increases around the public and private schools in the census tracts.
The paper continues with a brief literature review on the economic and social impact of fast-food restaurants. The following section presents the data descriptions and data generation process. Next, we provide a geographical analysis on the fast-food restaurant density, ethnicity (black and white neighbourhoods), and wealth (median household income) at census tract level. Section V discusses the model specifications for the econometric analysis. After the estimation, results are presented, the paper concludes with policy recommendations and a summary of key findings.
Literature Review
The existing literature on the economic and social impact of fast-food restaurants is divided into three main categories.
The first group focuses more on the relationship between fast-food intake and health status, particularly obesity and diabetes. Studies showed that there is a high correlation between fast-food intake and health status. In particular, fast food intake stimulates higher body mass index (BMI), weight gain, and development of insulin resistance, which are important risk factors for diabetes and obesity (French et al., 2000; Jeffrey and French, 1998; Edelstein et al., 1997). These studies concluded that higher prevelance of fast-food restaurants has been one of the leading cause for increasing unhealthy food consumption.
The second and third groups of the literature examine demographic indicators affecting geographical distribution of fast-food restaurants within a city and how they influence neighbourhood’s health condition (Yen and Kaplan, 1999; Diez-Roux et al., 1997; Stokols, 1992). The distribution of fast food restaurants within a city has a significant impact on the residents’ health condition because some studies proved that individuals’ health and behaviors are affected by their social and physical surroundings. They reported, for example, spatial proximity to a medical facility is positively related to utilization of healthcare. They also reported that living close to the places selling alcohol causes an increase in alcohol consumption.
Researchers have found the distribution of fast-food restaurants within a city is closely related to a variety of demographics, such as race, ethnicity, income and so forth (Massachusetts Medical Society Committee on Nutrition, 1999; French et al., 2000; Jeffrey and French, 1998). Among others, Reidpath et al. (2002), study the income and exposure to the fast food and suggest that fast-food restaurant density and median individual income are negatively correlated (they measured wealth as median individual weekly income). In a more comprehensive study, Jason et al. (2004) examine the relationship between the geographic distribution of fast-food restaurants, ethnicity, and income. They concluded fast-food restaurant density is positively correlated with non-white neighbourhood and negatively correlated with median household income. Additionally, Morland et al (2002) tested the link between the spatial density of fast-food restaurants, racial distribution, and income level. They found no consistent relationship between wealth and the location of fast-food restaurants (they measured wealth as a median house prices at a census tract).
The clustering of fast-food restaurants around public and private schools and their impact on students’ health condition is also discussed in the literature. Austin et al (2005), for example, examined the concentration of fast-food restaurants in areas proximal to the schools to characterize school neighbourhood food environment. They reported that fast-food restaurants are concentrated within a short walking distance from schools and exposing children to unhealthy food environments in their school neighbourhoods in Chicago, IL. Simon et al. (2008) studied on the proximity of fast-food restaurants to public schools and examined proximity by neighbourhood income and school level in Los Angeles County, CA. Their result suggests that fast-food restaurants are located in close proximity to many schools, especially high schools and schools located in low income highly commercial neighbourhoods. Davis and Carpenter (2009) also analyzed the relationship between fast-food restaurants located around the schools and obesity among middle and high school students in California. They concluded that students within a school located around fast-food restaurant consume more soda and fewer vegetables, and were more likely to be overweight or obese.
In this research, we aim to explore the link between the fast-food restaurant density and socioeconomic characteristics in the US metropolitan areas having high rates of obese population. In particular, we study Montgomery, AL which is among the most obese cities in the US (Gallup and Healthway Survey, 2009). We consider the demographic and socioeconomic characteristics of these cities along with the locational distributions of fast food chains. Our objective is to reveal whether the demographic and socioeconomic characteristics of a city affect the supply of fast food chains at the most disaggregated level (i.e., census-tract level). In addition, we investigate the effects of school locations on prevelance and density of fast food restaurants. Our research combines two strand of the relevant literature, namely, geographical and econometric analysis. In the geographical analysis, we combine demographic, economic, and geographical data for each census tract in the cities. In addition to this, geographical analysis allows us to obtain data for locational interrelations between fast food restaurants and school zones. Then, we estimate econometric models to explain the impacts of neighbourhood characteristics on fast food supply.
Data
Data generation process for the empirical analysis consists of two stages. First, socioeconomic data are obtained from US government databases at the census-track level for 2010. The data for median income and unemployment rates come from the US department of labour and the data for educational attainment and demographic factors come from the US census bureau. Geographic Information System (GIS) software is employed to derive geographical information which is combined with the socioeconomic data for further econometric analysis.
A comprehensive list of fast-food restaurants for Montgomery city is obtained from the city GIS department of Montgomery along with their street address and census tract identification codes. Demographic characteristics such as, population, race, and income are obtained from U.S. Census Bureau (www.factfinder2.census.gov) at the census tract level for the year 2010. Census tracts maps are acquired from 2010 Census TIGER/Line Shapefiles (http://www.census.gov/geo/www/tiger/tgrshp2010/tgrshp2010.html).
After collecting necessary data and GIS layers, all demographics and 340 fast-food restaurants are matched to 163 census tracts within Montgomery city limits by using GIS. In order to find fast-food restaurant density for every a thousand people in each census tract, the number of fast-food restaurants is divided by the total population in that census tract and multiplied by 1000. Finally, fast-food restaurant density, population, race, and income level of every census tracts are combined to analyse the associations between fast-food density and demographic and economic status (see map 1, 2, and 3).
GIS software allows us to analyse the locational patterns of fast-food restaurants and neighbour characteristics (e.g., schools) within Montgomery city limits. The compiled list of public and private schools is collected from city GIS departments along with their street addresses and census tract identification codes. After matching all fast-food restaurants and schools to each census tract of Montgomery cities, 400-m-radius and 800-m-radius buffers are created around 100 public and private schools. Then, the number of fast-food restaurants located within buffers of each size is calculated. The 400-m buffer zone is used in the current study because, on average, an adult can walk 400 m in 5 minutes. 800-m-radius buffers is created to characterize how the distance affects the school’s food environment (see map 4).
Geographical Analysis
The census tract map of Montgomery city in Map 1[1] shows both the density of fast-food restaurants as well as the percentage of black and white population. The first number above every fast-food restaurant symbols denotes the number of fast-food restaurants, and the second number on the symbols shows fast-food restaurant density in every census tract. The bars stand for the percentage of black and white residents in each census tract. 56 percent of population in Montgomery is black and 39 percent is white. The percentage of black population is higher than white population in 32 census tracts (called black census tracts in the current study) and vice versa for the rest 27 census tracts (called white census tracts in the current study) within Montgomery city limits (see Map 2 for detailed information about black and white census tracts). There are 170 fast-food restaurants in the black census tracts and the average density of fast-food restaurants in these tracts is 1.79. That’s mean there are approximately two restaurants for every a thousand people in black census tracts. The case is significantly different for white census tracts. The number of fast-food restaurants is 172 and the density is 1.42. There are less than one and half restaurants for every a thousand people in white census tracts.. This finding suggests that fast-food restaurants are densely located in predominantly black populated areas in Montgomery, AL.
The census tract map of Montgomery city in Map 3 shows both average household income level of the census tracts and the density of fast-food restaurants. The first numbers on the census tracts indicate the number of fast-food restaurants and the second numbers denote to average household income level of that census tract. Average household income is less than $34000 in 21 census tracts. There are 96 fast-food restaurants in these poorest census tracts and fast-food restaurant density is 1.94. In twenty census tracts, average household income ranges from approximately $34000 to $60000. 136 fast-food restaurants are located in these census tracts. Although the number of fast-food restaurants is higher in these tracts compared the census tracts with lower income level, the density of fast-food restaurants is less, which is 1.56. 15 census tracts have average household income level which ranges between $60000 and above. They have 110 fast-food restaurants and density of these restaurants is 1.30 which is less than previous census tracts groups with lower income level. These results indicate that fast-food restaurants are densely located within the low-income neighbourhoods. The results are in accordance with the common view in the literature which argue the population with low-income level more exposure to unhealthy foods emerge from fast-food restaurants.
The census tract map of Montgomery city in Map 4 reports both the density of fast-food restaurants as well as the spatial distribution of the schools included in analyses. Map 4 also indicates schools that have no fast-food restaurant or at least 1 fast-food restaurant within 400 or 800 m. A hundred public and private schools are analysed in the current study. Seventeen percent of total fast-food restaurants (61 restaurants) are located within 400-m-radius around schools. Fifty-seven percent of total number of fast-food restaurants in Montgomery city limits (193 restaurants) is placed within 800-m-radius of schools. 25% percent of schools in Montgomery city have at least 1 fast-food restaurant within 400 m, whereas nearly 65% of schools have at least 1 fast-food restaurant within 800 m, approximately a 10 minute walk. These statistics shows that students in Montgomery can easily reach a fast-food restaurant. These results may be one of the main indicators of high childhood and adult obesity rates in Montgomery city. According to Gallup and Healthway Survey in 2009, Montgomery city is ranked No. 1 among the most obese 187 metro areas in the U.S. with adult obesity rates of 34.6%, substantially surpassing the national average obesity rate of 26.5% (www.gallup.com). In addition, studies from Centers for Disease Control and Prevention ranked Alabama No. 14 in the nation for childhood obesity in 2010.
Econometric Analysis
This section explores the determinants of the fast food restaurant density by an econometric analysis. A number of models for univariate count data including Poisson, Negative Binomial, Zero inflated Poisson and negative binomial models are employed in the empirical work. In all models, the number of fast food restaurants (FF) is used as the dependent variable and three types of measures are included in the explanatory variables, which are number of schools, demographic data (race, gender), and socio-economic information (unemployment, Gini-index, educational attainment and median household income). Table 1 presents the summary statistics for the data.
Table 1. Descriptive Statistics, Dependent and Explanatory Variables | ||||||||
Variable | N | Mean | Variance | Min | Max | 25th Pctl | 50th Pctl | 75th Pctl |
N of FF Restaurants | 162 | 5.494 | 49.456 | 0 | 39 | 0 | 3 | 8 |
Median Household Income | 161 | 10.490 | 0.280 | 8.794 | 11.685 | 10.188 | 10.547 | 10.872 |
Black Alone | 162 | 0.507 | 0.107 | 0 | 1 | 0.21 | 0.445 | 0.82 |
N of Schools | 162 | 1.565 | 2.360 | 9 | 0 | 1 | 2 | 2 |
Unemployment | 162 | 11.434 | 79.090 | 0 | 46.1 | 4.8 | 8.55 | 16.3 |
B.S or More | 162 | 30.465 | 611.764 | 0 | 119.6 | 11.4 | 23.85 | 44.1 |
Some High School | 162 | 41.106 | 708.825 | 0 | 140.9 | 20.4 | 37.25 | 56.8 |
Female | 162 | 0.527 | 0.003 | 0.27 | 0.66 | 0.5 | 0.53 | 0.56 |
Gini_Index | 161 | 0.430 | 0.005 | 0.25 | 0.66 | 0.38 | 0.42 | 0.47 |
A good starting point of count data modelling is to illustrate the empirical distribution of observed counts by using the histogram. Figure 1 shows the frequencies of number of FF.
Figure 1. Distribution of the Dependent Variable: Number of FF Restaurants
To see the figure please download the article
Poisson regression is the basic model for count data and assumes that each observed count Yi is drawn from a Poisson distribution with the conditional mean ui on a given vector Xi for case i. Since it has a very strong assumption that is the conditional variance equals conditional mean. Data appropriate for Poisson regression do not happen very often. Nevertheless, Poisson regression is used as a starting point and a baseline for modelling the count data for fast food restaurants. Due to the high possibility of over-dispersion and excess zero problems we will consider the alternative approaches such as Negative Binomial and Zero inflated models.
Negative binomial regression can be used for over-dispersed count data; it has a similar structure with Poisson regression and has an extra parameter (Alpha) to model the over-dispersion. Zero-inflated regression models are trying to control for excess zero problems. There are two kinds of zeros are assumed to exist in the data, “true and excess zeros”. Zero-inflated models estimate two equations simultaneously, one for the count model and one for the excess zeros. As illustrated in Table 1, the variance of number of FF is about ten times of the mean, implying the possibility of over-dispersion, which means that the ratio of the conditional variance to the conditional mean is greater than one. A further screening on the data also shows that more than 25% of the census tracts, 43 out of 162, have no fast food restaurants, indicating excess zeroes.
First, the Poisson regression is used on the fast food data. All variables except number of schools are statistically significant. However, results are biased due to two major symptoms detected in the previous section. Under the Poisson model, the predicted proportion of zero fast food restaurants is 0.023, which considerably underestimates the observed proportion. Thus, the magnitude of standard errors decreased and t-statistics are inflated.
Table 2. Estimation Results for All Models | |||||
DF | Poisson | N.B | Z.I.P | Z.I.N.B | |
Intercept | 1 | 9.650***
(1.659) |
10.658**
(4.968) |
7.001***
(1.697) |
8.648*
(4.578) |
Hh income | 1 | -0.790***
(0.141) |
-0.903**
(0.429) |
-0.557***
(0.144) |
-0.670*
(0.396) |
Black | 1 | 0.108***
(0.028) |
0.360***
(0.126) |
0.348***
(0.050) |
0.389***
(0.116) |
Schools | 1 | 0.365***
(0.050) |
0.111
(0.083) |
0.122***
(0.030) |
0.110*
(0.067) |
Unemployment | 1 | -0.012
(0.008) |
-0.021
(0.019) |
-0.005
(0.008) |
-0.028
(0.018) |
Bachelors or more | 1 | 0.011***
(0.002) |
0.013*
(0.007) |
0.009***
(0.002) |
0.005
(0.006) |
Some high school | 1 | -0.003
(0.002) |
-0.006
(0.006) |
0.000
(0.002) |
-0.005
(0.005) |
Female | 1 | -2.012***
(0.714) |
-2.260
(2.087) |
-1.514**
(0.722) |
-3.824**
(1.942) |
Gini_Index | 1 | 1.202**
(0.577) |
2.218
(1.870) |
1.282**
(0.622) |
3.982**
(1.679) |
Inf_Intercept | -9.175
(10.444) |
-29.296
(27.759) |
|||
Inf_hh income | 0.739
(0.863) |
2.976
(2.543) |
|||
Inf_Black | -0.029
(0.261) |
-0.152
(0.748) |
|||
Inf_Schools | -0.068
(0.165) |
0.415
(0.287) |
|||
Inf_Unemployment | 0.035
(0.035) |
-0.111*
(0.093) |
|||
Inf_Bachelors or more | -0.015
(0.013) |
-0.195**
(0.084) |
|||
Inf_Some high school | 0.011
(0.011) |
-0.012
(0.025) |
|||
Inf_Female | 2.253
(3.890) |
-18.063*
(10.424) |
|||
Inf_Gini_Index | -2.753
(3.723) |
23.722**
(11.186) |
|||
_Alpha
Predicted Zeros (%) AIC SBC Number of Obs |
0.023 1310 1337 154 |
1.171***
(0.177) 0.221 835.317 865.687 154 |
0.256 1073 1128 154 |
0.835***
(0.153) 0.237 834.912 892.614 154 |
Note: Numbers in parenthesis are the standard errors for the parameter estimates.
***,**,* Statistical significance at 1%,5%, and 10% levels, respectively.
Negative binomial regression addresses the issue of over-dispersion by including a dispersion parameter to accommodate the unobserved heterogeneity in the fast food data. The negative binomial more closely estimates the proportion of zeros (0.221). Also, the test of the dispersion parameter, Alpha, in the negative binomial model indicates significant over-dispersion. Finally, the AIC and BSC values are significantly improved in the NB model. As a result, the negative binomial model is preferred to the Poisson model.
Another way to account for the large number of zeros in this data set is to fit a zero-inflated Poisson (ZIP) or a zero-inflated negative binomial (ZINB) model. The coefficient significance in the Logit component suggests that whether a restaurant is occurred in the census tract depends on explanatory variables. While, the Poisson component indicates that the frequencies of fast food restaurants are related to independent variables. The first set of parameters gives the estimates of in the model for the Poisson process mean. Parameters with the prefix “Inf” are the estimates of Gamma in the logistic model for .
The proportion of zeros predicted by the ZIP model is 0.256, which is much closer to the observed proportion than the Poisson model. However, Figure 2 shows that it significantly deviates from the observed proportions for the values greater than one restaurant.
Estimated coefficients of all four models together with related statistics are listed in Table 2. While Poisson regression provides a baseline model for fast food data, the other three suggest the better fit than the basic Poisson model. As is seen in Figure 2, ZIP model significantly dispersed from the observed probabilities for the number of restaurants around 1 and 9, we rely on the estimated coefficients on ZINB and NB. However, all models fit in a similar way for the number of fast food restaurants bigger than 15.
Figure 2. Average Predicted Count Probabilities for Models
To see the figure please download the article
Furthermore, Vuong test results for Poisson model rejects the null hypothesis that models are equally close to the true model, thus Zero Inflated Model is preferred over Poisson. However, it is stated that ZINB preferred over the negative binomial model according to Vuong test.
Table 3. Vuong Test Results: ZIP vs. Poisson, ZINB vs. NB | |||
Vuong Statistic | Z | Pr>|Z| | Preferred Model |
Unadjusted | 1.688 | 0.091 | zinb |
Akaike Adjusted | -0.172 | 0.863 | zinb. |
Schwarz Adjusted | -3.032 | 0.002 | neg. bin. |
Unadjusted | 4.424 | <.0001 | zip |
Akaike Adjusted | 4.243 | <.0001 | zip |
Schwarz Adjusted | 3.965 | <.0001 | zip |
Results
Four count data models are tested for compatibility. Although zero inflated model is the best predictor for excess zero values, it has biased prediction for the range of fast food restaurants between 1-10. Besides, since the favourable vuong test results and the significant alpha parameter in the zero inflated negative binomial model which indicates the over-dispersion is both caused from the unobserved heterogeneity and splitting structure, here, we limit the discussion with the results of ZINB regression model, which include a logit and a NB regression.
The basic setup of the logit model is to predict the likelihood of prevalence of fast food restaurants in the census tracts. In the logit model, the coefficients for the Gini-index, percentage of having Bachelor’s or more, females, and unemployment variables are statistically significant at 1%, 5%, 10%, and 10% levels, respectively. Income inequality is positively correlated with both the likelihood and frequency of fast food restaurants. While the percent of unemployment, females and having bachelors or more degree decrease the likelihood of the occurrence by a factor of 0.895 and 0.823, respectively. However, fast food frequency is similar with other levels of educational attainment and employed people.
The NB regression part also suggests that log of median household income decreases the number of fast food restaurants versus the number of schools increases the fast food density in the census tracts. The percent of the black population and the income inequality are the most statistically significant factors that trigger the frequency of the fast food restaurants by 0.678 and 0.672 in the order of appearance.
Conclusions
We examine the link between prevelance of fast-food restaurants and economic and social characteristics in the US metropolitan areas struggling with obesity. Our objective is to reveal whether the demographic and socioeconomic characteristics of a city affect the supply of fast food chains. In this respect, we study Montgomery, AL which is among the most obese cities in the US (Gallup and Healthway Survey, 2009). We consider the demographic and socioeconomic characteristics of the city along with the locational distributions of fast food chains to explore how the supply of fast food restaurants is distributed in the Metropolitan areas by using geographical information systems and count data models at the most disaggregated level (i.e., census-tract level). The study is also extended to analyse the effects of school locations on prevelance and density of fast food restaurants. Thus, all public and private schools in the city limits of Montgomery are analysed in the current study.
The empirical findings of this study suggest that neighbourhood, both demographic and economic, characteristics have significant impact on fast food supply. Among others, the ethnicity and income inequality are the most important factors on prevelance and density of fast food restaurants. Model estimates show that the fast-food restaurant density is higher in neighbourhoods with predominantly black population and higher income inequality. Results further indicate that the number of the fast food restaurants increases around the public and private schools in the census tracts. Geographical analysis results indicate that 25 percent of schools in Montgomery city have at least 1 fast-food restaurant within 400 m, whereas nearly 65% of schools have at least 1 fast-food restaurant within 800 m, approximately a 10 minute walk.
These results can be possible indicators of high childhood and adult obesity rates in Montgomery city along with the key determinants of overweight and obesity prevalence for adults and children suggested in the literature. According to Gallup and Healthway Survey in 2009, Montgomery city is ranked No. 1 among the most obese 187 metro areas in the U.S. with adult obesity rates of 34.6%, substantially surpassing the national average obesity rate of 26.5%. 2010 CDC (Centers for Disease Control and Prevention) report ranked Alabama No. 14 in the nation for childhood obesity.
Appendix
Figure 3: Fast-Food Restaurant Density and Race in Montgomery, AL, 2010
To see the figure please download the article
Figure 4: Black And White Neighbourhoods and Fast-Food Restaurant Density in Montgomery, AL, 2010
To see the figure please download the article
Figure 5: Median Household Income Level and Fast-Food Restaurant Density in Montgomery, AL, 2010.
To see the figure please download the article
Figure 6: Location of Schools and Fast-Food Restaurant Density in Montgomery, AL, 2010
To see the figure please download the article
References
Austin, S. B., Melly, S. J., Sanchez, B. N., Patel, A., Buka, S., & Gortmaker, S. L. (2005). Clustering of fast-food restaurants around schools: a novel application of spatial statistics to the study of food environments. American journal of public health, 95(9), 1575-1581.
Block, J. P., Scribner, R. A., & DeSalvo, K. B. (2004). Fast food, race/ethnicity, and income: a geographic analysis. American journal of preventive medicine, 27(3), 211-217.
Centers for Disease Control and Prevention. Available at : http://www.cdc.gov/obesity/ childhood/ (Accessed August 12, 2017)
Critser, G. (2004). Fat land: How Americans became the fattest people in the world. Houghton Mifflin Harcourt.
Davis, B., & Carpenter, C. (2009). Proximity of fast-food restaurants to schools and adolescent obesity. American Journal of Public Health, 99(3), 505-510.
Diez-Roux, A. V., Nieto, F. J., Muntaner, C., Tyroler, H. A., Comstock, G. W., Shahar, E., … & Szklo, M. (1997). Neighbourhood environments and coronary heart disease: a multilevel analysis. American journal of epidemiology, 146(1), 48-63.
Edelstein, S. L., Knowler, W. C., Bain, R. P., Andres, R., Barrett-Connor, E. L., Dowse, G. K., … & Collins, V. R. (1997). Predictors of progression from impaired glucose tolerance to NIDDM: an analysis of six prospective studies. Diabetes, 46(4), 701-710.
French, S. A., Harnack, L., & Jeffery, R. W. (2000). Fast food restaurant use among women in the Pound of Prevention study: dietary, behavioral and demographic correlates. International Journal of obesity, 24(10), 1353.
Jeffery, R. W., & French, S. A. (1998). Epidemic obesity in the United States: are fast foods and television viewing contributing?. American journal of public health, 88(2), 277-280.
Lin, D. B. B. H. (1999). Away-from-home foods increasingly important to quality of American. Agriculture Information Bulletin No, 749.
Massachusetts Medical Society Committee on Nutrition. (1989). Fast-food fare. North England Journal of Medicine; 321:752–6.
Morland, K., Wing, S., Roux, A. D., & Poole, C. (2002). Neighbourhood characteristics associated with the location of food stores and food service places. American journal of preventive medicine, 22(1), 23-29.
Reidpath, D. D., Burns, C., Garrard, J., Mahoney, M., & Townsend, M. (2002). An ecological study of the relationship between social and environmental determinants of obesity. Health & place, 8(2), 141-145.
Schlosser, E. (2002). Fast food nation: What the all-American meal is doing to the world. Penguin UK.
Series, T. F. S. T. (2004). Limited Service Restaurants (1975–2005). Chicago Ill Technomic Inc.
Simon, P. A., Kwan, D., Angelescu, A., Shih, M., & Fielding, J. E. (2008). Proximity of fast food restaurants to schools: do neighbourhood income and type of school matter?. Preventive medicine, 47(3), 284-288.
Stokols, D. (1992). Establishing and maintaining healthy environments: toward a social ecology of health promotion. American psychologist, 47(1), 6.
United States Census – Database. Available at: http://www.census.gov/geo/www/tiger/tgrshp2010 /tgrshp2010.html (Accessed May 12, 2017)
Yen, I. H., & Kaplan, G. A. (1999). Neighbourhood social environment and risk of death: multilevel evidence from the Alameda County Study. American Journal of Epidemiology, 149(10), 898-907.
[1] All the maps generated in this study presented in the appendix section.