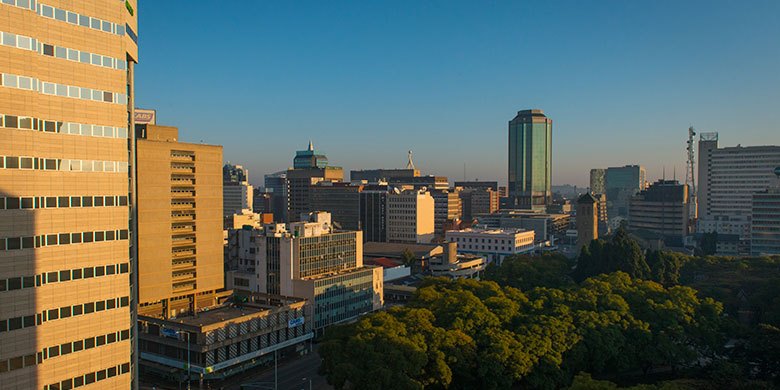
Abstract
This study examined the role played by bank specific factors in explaining bank stability in Zimbabwe. A panel data set was compiled covering 15 CAMEL type ratios from all 26 banking institutions which were operational in Zimbabwe between 2009 and 2014. The Principal Component Analysis (PCA) and Random Effects (RE) model were applied to establish the statistically significant CAMEL type bank distress prognosticators in Zimbabwe. This study revealed that Capital, Asset Quality and Earnings (CAE), are the most statistically significant bank specific factors which influence distress in the Zimbabwean market. On the macroeconomic front, statistical significance was established on the ‘end-period Consumer Price Index (CPI)’ in determining bank stability in Zimbabwe. This implies that a deteriorating asset quality base in a bank with a feeble capital base and weak earnings in a market dogged by inflation instability is a classic precursor to bank distress in Zimbabwe.
Key words: Bank stability, Bank specific factors, Principal component analysis, Panel data analysis
JEL Classification: G01, G21, C58
To Download click here.
How to Cite:
MUPARURI, A. and AKINSOLA, F. (2018), ‘Explaining Bank Stability Using Bank Specific Factors in Zimbabwe’, Journal of Global Analysis, 8(1): 45-72.
Introduction
Globally, economic development is highly influenced by the state of the financial sector. Instances of bank instability, such as that witnessed during the 2007-2009 Global Financial Crisis (GFC), are very costly to the affected economies (or even the global economy at large depending on the magnitude of occurrence). Several episodes of bank instability have been observed across major financial jurisdictions in the past. These episodes of financial distress have always resulted in the regulatory authorities crafting responsive regulatory frameworks to counter the root cause of the crises hence preventing further crises of similar nature.
Regulatory authorities endeavour to stabilise banks through the use of both macro prudential and micro prudential regulatory toolkits. The former entails setting up of policies which strengthen the banking sector at a macro level, whilst the latter, acting on a supportive basis, encompass the implementation of a surveillance mechanism, which focuses on the strengthening of individual banking institutions (BIS, 2001). Regulatory authorities in various market jurisdictions tend to converge on the use of the CAMELS rating system when conducting onsite and offsite individual bank examinations.
Meanwhile the African continent faces a unique developmental crisis. Being the poorest region in the world, it is also the only major developing region in the world which experienced negative growth between 1980 and 2000 (McArthur, Schmidt-Traub, Kruk, Bahadur, Faye & McCord, 2004:117). The negative growth caused significant distress in the underlying financial markets.
Idiosyncratically, the Zimbabwean banking sector experienced heightened bank distress over the past decade especially among the second and third tier banking institutions. This study seeks to establish the endogenic factors which affect bank stability within the market. The study further seeks to build a bank distress prediction model which will bolster the current early warning signals to bank distress in Zimbabwe and the region.
In 1985, the Zimbabwean banking sector had a total of twenty institutions, mainly dominated by oligopolistic players in the form of expatriate and Government related banks. As shown in Table 1 below, the number of players in the Zimbabwean banking sector increased dramatically after financial sector liberalisation in the 1990s, before dwindling to below their starting point within two decades.
Plotting the total number of institutions against time on a scatter graph will produce a graph which mimics a ‘U’ shaped curve. This development suggests that the banking sector in Zimbabwe has experienced a nexus of changes in a frenetic manner. Earlier changes showed progressive signs as the sector shadowed the developments on the global financial landscape.
The hegemony of bank distress over the past decade in Zimbabwe may point to a financial crisis. The statistics on the profile of banks depicted in Table 1, speak to the underlying financial instability in the country. A disturbing observation though is that bank distress seems to be more prevalent in the second to third tier banks, which are mainly indigenously owned. This background of a non-ubiquitous pattern in bank distress pattern warrants an investigation of the current micro prudential policy framework in the country.
The RBZ widely uses the CAMEL(S) framework when conducting their onsite and offsite bank examinations. Several questions can be put forward on the causality, predictability, and possible remediation of such a catastrophe. Numerous hypotheses can be put forward to try and demystify the lacuna of information inherent in this status quo. This research endeavours to establish the main bank specific financial indicators which influence bank stability, and to build a mathematical model (probabilistic) which can predict financial distress in Zimbabwean banks. The research will be premised on the following objectives;
- To determine the level of influence of various CAMEL(S) type bank specific factors in explaining bank distress in Zimbabwe.
- To build a mathematical model which can forecast bank distress in Zimbabwe using the CAMEL(S) type bank specific factors.
In order to interrogate these objectives, the following research questions will be asked;
- Which CAMEL(S) type bank specific factors attribute to bank distress with statistical significance in Zimbabwean banks?
- Can the likelihood of bank distress in Zimbabwe be predicted with absolute certainty?
The Zimbabwean Banking Sector – An Overview
The Zimbabwean financial sector has also been dogged by episodes of financial distress. The collapse of ENG Capital in 2004 can be viewed as the trigger event in the financial crisis that ensued in the country in the new millennium. Figure 1 below depicts the number of banking institutions by institution type which have experienced financial strife in the past decade.
Figure 1: Distressed Banks in Zimbabwe (2004-2015)
From Figure 1 above, it is conclusive that liquidations have been rampant, affecting a total of thirteen banks during the period. Five banks are currently under recuperative curatorship; whilst two are currently being classified as ‘troubled’ by the central bank. This brings the total to twenty banks which have experienced distress at various magnitudes over the past decade.
The distress in the financial service sector has eliminated virtually all locally owned financial institutions. Steward bank is the only locally owned bank which arguably may be deemed to be stable and still operational in the country, although it is not a Systematically Important Financial Institution (SIFI) due to its low deposit base, as well as weak profitability position. The resilience of Steward bank may be attributed to its strong shareholder (Econet Wireless Limited) who has managed to fully capitalise the bank. Other formerly indigenous banks such as Banc ABC and NMB Bank have sold off controlling shareholding stakes to foreign shareholders in varying schemes of arrangement.
From an interest rate perspective, there has been a huge disparity between savings and lending rates in the banking sector since the introduction of the multicurrency regime in 2009; the main interest rates which prevailed in the country are illustrated in Figure 2 below. Savings attracted no return in the market between 2009 and 2010. The average market rate however crept upwards to its current high of 3.4% in 2014.
Figure 2: Average Market Interest rates in Zimbabwe (2009-2014)
On the contrary lending rates have been relatively high throughout the spectrum. The downward shift in the nominal minimum lending rate from 15% in 2011 to 6% in 2013 was met by an opposing upward shift in the average nominal maximum lending rate. The result of this is that the nominal average lending rate has remained oscillating between 20% and 25% since 2009. When mapped against the savings rates these lending rates reflect higher margins being applied to the cost of funds by banks across the sector.
The high disparity between lending and deposit rates in the country signals the heightened level of liquidity shortage in the market, hence the huge liquidity premium. Such huge premiums are unsustainable in the normal course of business. Finance theory attests to the concept of moral hazard which may drive the riskier clients to take up the expensive loans. These riskier clients will ultimately default on their obligations. This is known as adverse selection on the bank’s credit portfolio and it appears to have been rife in the Zimbabwean banking sector over the past six years as shown by the increase in Non-Performing Loans to advances (NPLs) in the sector.
The upward trajectory in the aggregate NPL levels denotes heightening credit risk levels in the economy. This comes amidst a hamstrung liquidity base in the country and it remains an Achilles heel in the stability of Zimbabwean banks. From a zero base in 2009, the NPL ratio peaked at 20% in September 2014 (RBZ, 2015). This unpleasant situation has prompted the central bank to create a special purpose vehicle called ZAMCO1 in 2014 to acquire and house corporate NPLs from banks under a predetermined criterion.
On the retail loan portfolios, the absence of a centralised and robust credit reference function in the country has heightened the possibility of an unsustainable household debt. In response to this problem, banking sector players have tried to mitigate this risk by restricting consumer loans to individuals who receive their salaries through the bank.
Literature Review
The differentiation between stable and unstable banks is a crucial policy issue in respect of early warning signals (EWS) of bank distress (Moyo et al., 2014). Firstly, it allows stakeholders to take recuperative action on time hence preventing a deeper crisis; this will invariably decrease the amounts needed in fiscal bail outs to restructure and recapitalise failed institutions (Maghyereh & Awartani, 2013).
Additionally, early prediction of fragility allows regulators to be better prepared for a catastrophe, hence affording them the opportunity to better manage and coordinate their efforts. Depending on the scale of occurrence, a bank crisis can destabilise other economic variables, such as the currencies market. This inadvertently weakens the economy and magnifies the distress cost (Maghyereh & Awartani, 2013).
Theoretical Framework
The practical application of macroeconomic variables in bank distress predictive models has not significantly improved accuracy of results (Quagliariello, 2008:106). This could be explained by the high predictive capability of indicators from specific financial statements if they are accurate and reliable. Therefore regressions run on bank specific factors tend to outperform those based on macroeconomic variables (Quagliariello, 2008:106). It is crucial to note that when the economic conditions in a country worsen, these may trigger heightened systematic risk, which naturally destabilises banks.
Numerous scholars (such as Mannasoo & Mayes, 2009; Baki, 2013; Maghyereh & Awartani, 2013; Karminsky & Kostrov, 2014; Quagliariello, 2008; and Arabi, 2013), agree on the relevance of macroeconomic factors in explaining bank stability. Jordan and Rosengren and Glogova et al. (as cited by Quagliariello, 2008:107), conducted a study to establish the significance of regional economic and macroeconomic variables in improving the ratings provided by the CAMELS rating model. Their study concluded that the inclusion of macroeconomic fundamentals adds little value in the assessment of the health of individual banks in the short term. The relevance however, increases if the forecast period is lengthened to at least four quarters.
Considerable work covering both macro prudential and micro prudential prescriptions to stabilise banks has been carried out in various geographical jurisdictions over the past years. The thrust of these developments is aimed at bolstering the resilience of financial institutions globally. This has resulted in the promulgation of committees such as the BCBS and the FSB (Bollard, 2011).
The Bank of Greece (2008) cites three fundamental causes of bank performance in current empirical and theoretical debates. They cite financial sector reforms, the level of market competition, and the risk affinity of individual banks as the key causative factors of bank performance. The first two variables relate to the macro prudential banking environment, whilst the latter one pertains to the eccentric risk pattern of a particular institution.
It is crucial to appreciate that banks are driven by competition, innovation and profitability (Petitjean, 2013). Most regulators in the world premise their risk management toolkits around the assumption that banks can be made less competitive or less innovative. This is not pragmatic, as competitive forces cannot be suppressed (Petitjean, 2013). Indeed, regulation can exert an external shock on the banking sector, which can lead to sub-optimal performance of institutions (Bikker & Bos, 2008).
Deposit Insurance Schemes (DIS) are common in most markets across the globe. The adoption of Deposit Insurance Schemes in financial markets can lead to an increased probability of bank failure. This is so because, on one hand depositors are less motivated to monitor bank performance, whilst on the other hand bankers tend to invest in riskier projects in order to offer higher returns to clients as a competitive edge. The deposit insurance tool, good as it may be, aggravates the moral hazard problem in banking (Karminsky & Kostrov, 2014; Haq & Heaney, 2011).
On the micro prudential level, academics have proposed several micro prudential oriented theories to demystify the causality of bank distress and its prediction thereof. Karminsky and Kostrov (2014), elucidate that the bank’s balance sheet structure, coupled with other financial characteristics of banks such as capitalisation levels, as well as bank size, are among the most important prognosticators of bank distress.
In as much as the financial intermediation role played by banks is crucial for economic development, this process however inadvertently creates liquidity and default risks for banks (Alhassan, Kyereboah-Coleman & Andoh, 2014). The bank’s liquidity position is a very crucial indicator of bank distress. Asset quality is a key factor in explaining bank distress (Maghyereh & Awartani, 2013). Poor asset quality normally manifests itself in increased NPLs as well as heightened ex-post provisioning levels, thereby grossly affecting the bank’s earnings (Louzis, Vouldis, Metaxas, 2011). Torna (2010, as cited by Maghyereh & Awartani, 2013) after analysing the 2007-2009 GFC observes the relevance of a bank’s asset portfolio in explaining stability.
The aforementioned bank specific distress factors have been observed and applied in the investigation of distress in various regions, most of them tailored for idiosyncrasies of specific regions. In 1979, in response to the need to universalise the rating and assessment criteria, the Federal Reserve recommended the adoption of a Uniform Institution Rating System (UFIS). The aim was to create a uniform platform for assessing the health status of banks (Christopoulous, Mylonakis & Diktapanidis, 2011:11). This system was later known as the CAMEL system in the early 1980s. The CAMEL(S) system’s thrust is to assess the viability of banks using five (now six) critical areas that focus on the bank’s performance and operations (Gupta, 2014:94).
Empirical Literature
Macroeconomic factors determine when distress is likely to occur in the banking sector at a higher level (Mannasoo & Mayes, 2009). Various studies have been undertaken to empirically prove the significance of macroeconomic factors in bank stabilisation (Baki, 2013; and Corsetti, Pesenti & Roubiniet, 2001). Empirical evidence has however, been mixed on the influence of macroeconomic factors on bank stability, (Athanasoglou, Brissimis and Delis (2008); Zaki, Bah and Rao (2011:318) and Nuxoll (2003:13).
Prior to the 2007-2009 GFC, sentiment was rife amongst academia and practitioners that broader safety of the banking environment could be preserved by fostering the individual safety of banks (Claessens, Evanoff, Kaufman & Kodres, 2011). Interpretations of the events surrounding the 2007-2009 GFC reveal that the ‘micro prudential’ regulation is no longer sufficient to ensure sector-wide stability due to the intricacies embedded in the complex networks of advanced financial markets which create systematic risk (Claessens et al., 2011).
The Dodd-Frank Wall Street Reform and Consumer Protection Act of 2010 in the US have both recognised this notion, and in response it has constituted a Financial Stability Oversight Council and mandated it to monitor and manage market systematic risk (Claessens, et al., 2011). In as much as it is widely envisaged that macro prudential regulation is the way to go in order to foster stability in the US banking sector, the purported causes of the ‘failure of micro prudential’ regulation have not been probed (Claessens et al., 2011).
Competition amongst financial institutions is healthy for the overall development of the market. (Barth et al. (2001:2) and Beck, Demirgüç-Kunt and Levine (2004:3)) all corroborate on the position that countries with regulatory authorities who thwart competition are more likely to experience financial instability.
Micro prudentially, (Gunsel (2010:92); Huang, Chang and Liu (2010:557) and Bessler & Kurmann (2014:165); Maghyereh and Awartani (2013:142) and Athanasoglou et al. (2008)) in separate studies all identified with significant statistical relevance, the influence of bank specific and other CAMEL type factors such as the bank’s liquidity position, asset quality, the structure of underlying loans2, net interest margin, the net equity base and credit risk on bank stability.
With regard to the African continent, (Tefula and Murinde (2002:4); Alhassan et al. (2014:60) and Adeyeye et al. (2012:166) established with huge statistical significance in the explanatory power of asset quality, liquidity, non-performing loans, capital adequacy, credit risk, and profitability levels, as well the commensurate liquidity level within the organisation, as the key financial variables which determine the health of banks.
The collapse of Lehman Brothers Holdings in 2008 had a major impact across several economies around the globe. Christopoulos et al. (2011) conducted a study to establish if the collapse could have been predicted using the CAMELS factors. The results clearly espouse that both the regulators and credit-rating agencies defied the logic in the CAMELS factors. They concealed the underlying problems which were glaringly obvious in all the CAMELS type variables, save for their liquidity indicators, which provided inconclusive evidence on the state of the bank.
The CAMELS model has also been applied to assess the health of public sector banks in India (Gupta, 2014:94). The results showed significant statistical differences in the analysed data, thereby validating the effectiveness of the CAMELS rating system. The improvement in the global bank supervisory framework has persuaded regulators to adopt the uniform rating framework (CAMELS Rating) in bank assessments (Gupta, 2014:102).
The World Bank (2010), writing in a policy research working paper on Jordan brings a fundamental twist to the appreciation of bank stability. The bank adds the need to assess corporate vulnerability in the country and its impact on the soundness of a bank, because the two are intimately interwoven. They indicate that systematic shocks to borrowers will be transmitted to banks through corporate defaults, thereby invariably affecting the stability of banks. They recommend incorporating corporate stress tests in the bank’s credit portfolio assessment in order to get accurate results from bank stress tests.
This background has influenced this research work, which aims to investigate the specific CAMELS type factors which influence distress in Zimbabwean banks.
Methodology
Defining Banking Distress
In the development of a probability of distress model the definition of distress is normally the initial step (Karmisky & Kostrov, 2014). The definitions of banking distress among academia and industry practitioners are varied, with generally acceptable definitions being premised on input from financial statements of the banks (Huang, Chang & Liu, 2010).
Hutchison (2002) defines banking distress as a multi-dimensional episode, whose impact varies from a minor to a large scale disruption. The isolation between minor and large scale disruptions is however not concise, although some considerable amount of work has been done to demarcate the two.
To make the distinction between distressed and stable banks more concise, the study made use of the IMF guidelines on Financial Soundness Indicators (FSIs) which were promulgated in 2006. FSIs have generally been adopted by practitioners to assess financial stability across markets. FSIs for the real, corporate, household, external, and financial sectors, as well as the financial markets were put forward. The International Finance Corporation (IFC, 2009:369) summarises the key measures of financial markets’ stability to change in equity, house prices, volatility, market liquidity and corporate bond spreads.
Assessing these variables in the context of this study leaves two variables eligible within the context of the financial ratios data set under review. These two variables are: change in equity and the liquidity indices. It is important to note that banking institutions with marginal capitalisation are more susceptible to liquidity risk as they lack sufficient liquidity buffers to absorb both exogenous and endogenous shocks (RBZ, 2014).
Given the differences in magnitude of bank distress or banking crises and the interrelatedness between liquidity and capital, this study jointly adopts the two commonly used FSIs in determining distress, which are the Liquid Asset Ratio (LAR) and the Capital Adequacy Ratio (CAR). This implies that a bank has to meet the minimum regulatory threshold on both ratios to be deemed stable. The RBZ enforces a minimum regulatory CAR of 12% and an LAR of 30% (RBZ, 2014). Levels below the minimum levels are considered distress episodes and they warrant a distress warning from the regulators.
Dependent Variable
The dependent variable in this study is bank distress. All periods where banking institutions jointly had a CAR lower than 12% and/or an LAR below 30% were considered to be distressed. The value of the dummy variable is ‘1’ for distress episodes, while ‘0’ is the contrary.
Explanatory Variables
Explanatory variables are financial ratios which were computed to assess the stability of banks. These ratios have been articulated after an extensive review of interrelated literature alongside consultations with other experienced researchers and industry practitioners.
Table 2 below synopsises the various ratios which denote the bank specific factors which were computed, and which formed part of the initial variables to be used in the modelling process.
Control Variables
Whitaker (2009) proposed that more firms get into financial distress as a result of poor management compared to economic distress. In order to put control measures for this notion in place, it is prudent to add other exogenous factors which can influence the association between bank distress and the bank specific financial indicators. These macroeconomic factors can affect the stability of a bank regardless of the soundness of specific financial soundness indicators (Whitaker, 1999). The GDP growth, end-period CPI and the bank’s average lending rates in Zimbabwe were utilised as control variables in this study. These statistics were gathered for the period between 2009 and 2014.
The population and sample
A balanced panel data set covering the 26 banking institutions which were operational in Zimbabwe between 2009 and 2014 was collated from publicly available secondary sources. Data was primarily obtained from the TFS Management Company’s financial database. The data was compared for accuracy using the stratified sampling technique with data from the RBZ website where it was available.
Principal Component Analysis (PCA)
PCA was utilised to reduce the dimensionality of the data, hence generating a parsimonious measurement of the data. The concept also shows the most important influences from numerous variables at the same time (Brooks, 2014:170). Additionally, this concept manages endogeneity in a data set and eliminates multicollinearity.
PCA is a statistical technique which uses an orthogonal transformation to change a set of possibly highly correlated variables into principal components, which are a set of linearly uncorrelated variables. Illustratively, if the original explanatory variables are symbolised by x1, x2, x3…., xn, the principal components can be denoted by p1, p2, p3…… pn. The principal components are independent linear combinations of the original variables (Brooks, 2014:170).
The principal components may be represented by the following set of equations;
P1 = α11 + α12x2 +….α1nxn
P2 = α21x1 + α22x2 +…α2nxn
Pn = αn1x1 + αn2x2 +…αnnxn………………………………………………….Equation 4.1
The PCA technique was employed to sort the 15 bank specific independent variables into 5 PCs with each PC better explaining the individual elements of the acronym CAMEL. The decision was reached after an analysis of the autocorrelation function between the principal components and the original independent variables to determine which principal component would better explain which CAMEL type variables. 3 control variables were added to the 5 PCs to arrive at 8 variables to be used for the modelling process.
A hypothesis test with the following null hypothesis was carried out when performing this test.
H0: The independent variables are free from multicollinearity and heteroscedasticity.
This process enhanced the sanity of the data set, and the remnant factors were used as input factors in the regression based model that was constructed.
The Modelling Process
The Regressors
The adopted 5 principal components were added to the 3 control variables and were used as input variables in the regression model. The random effects model was run on the data.
The Random Effects (RE) Model
The RE model is premised on the assumption that the variation across entities is assumed to be random and uncorrelated with the explanatory variables. It is utilised when there is reason to believe that the endogenous differences across entities have some influence on the dependent variable (Torres-Reyna, 2007:25). The estimation equation is largely similar to the FE model, save for the error term, which is split into two and is shown below:
Yit = αi + β1Xit+µit +ԑit……………………………………………….…………… Equation 4.2
Where,
Yit= the dependent variable where I denotes the entity and the t denotes time.
αi = this is the unknown intercept for each entity. The ‘I’ will take values between 1 and n.
β1= the coefficient for the explanatory variable.
Xit = Represents one explanatory variable at time t.
µit = between entity error term
Distress Prediction
Output from the regression analysis was used to produce a Z score which was used as input in the construction of a bank distress prediction model in Zimbabwe. The logit function was employed to construct the distress prediction function.
The Logit regression model attempts to find the values of β0 and βi’s which minimise the error in the value of y. The logit function is expressed by equation 4.3 below:
PD = 1 / (1+ exp( – y))…………………………………………..Equation 4.3
This function provides the probability of bank distress with values between 0 and 1.
Results and Discussion
Using the PCA concept and random effects model, this study estimates the CAMEL type determinants of bank distress in Zimbabwe during the period between 2009 and 2014. The logit function was further applied on the Z score from the output of the RE model to predict the probability of bank distress in Zimbabwe.
The DATA Set
After the collection and collation of data on the 26 banks which were operational between 2009 and 2014, 15 CAMEL type ratios shown in Table 4.1 were calculated for all the banks spanning over the 6-year period. Of the 26 banks operational in Zimbabwe during the analysis period, 7 had at least 4 out of 6 years of data missing. These banks were not reporting consistently due to various reasons, including bank distress.
Three macroeconomic control variables namely ‘end-period’ Consumer Price Index (CPI), Gross Domestic Product (GDP) growth rate and the Nominal Average Lending Rate (NALR), sourced from the Zimbabwean Central Statistical Office (CSO) were also incorporated into the model as control variables.
Data analysis was performed using the statistical software package STATA 11. An autocorrelation test of the 15 explanatory variables was computed and the matrix exudes high levels of multicollinearity across variables, with 5 pairs of ratios with an autocorrelation matrix above 80%.
PCA was utilised to deal with the multicollinearity problem within the data. This technique re-arranges components into linear combinations which are free from multicollinearity (Brooks, 2014:171), as shown in Table 3 below:
A benchmark of 80% adopted from the proposition of Koop (2009) was used to delineate between high multicollinearity amongst variables. The autocorrelation matrix in table 3 above show results which are much lower than the 80% guideline. This validates the acceptance of the null hypothesis in section 4.5 above and concluding that the data set was free from multicollinearity and heteroscedasticity.
Secondly, the PCA technique reduced the dimensionality of the data set under study. From the 15 PCs, 5 principal components (which individually better explain each variable of the acronym CAMEL) were chosen, based on their alpha values as shown in Table 4 below.
The results conform to the concept of parsimony, which is one of the hallmarks of good modern research. The observed data was transformed using the equations below to arrive at 114 principal components of each CAMEL factor.
PC15k=+α1CARij+α2LVRi……………………………………………………………………………Equation 5.1
PC13k=+ α1NPLij+ α2NPLAij+ α3IARij………………………………………………………..Equation 5.2
PC6k=+ α1BERij+ α2AURij+ α3SCTCij…………………………………………Equation 5.3
PC1k=+ α1ROEij+ α2ROAij+ α3NIMij …………………………………………Equation 5.4
PC10k=+ α1LARij+ α2LDR1ij+ α3LDR2ij+ α4FARij…………………………Equation 5.5
For all i=1… 19; the number of banks observed
For all j=1… 6; the number of years of observations
For all k=1… 114; the total number of all observations.
An analysis of PC15 reflects that both CAR and LVR have almost equal magnitude contribution towards PC15 but with an inverse relationship, that is an increase in CAR implies an increase in PC15, and an increase in LVR mean a decrease in PC15. Similarly, PC13 is greatly determined by NPL and NPLA while IAR is highly insignificant with an alpha value of 0.0119.
Results Analysis
The Regression Process
The RE model was run and the results are summarised in Table 5 below:
After the regression process, a Hausman test was run to validate the suitability of the RE model. This test is meant to qualify whether the unique errors µit exhibit some multicollinearity with the explanatory variables Xit or not. The HT results depicted the Prob>Chi2 at 0.7848. This value is insignificant at the 5% significance level, therefore H0 is accepted, and the researcher concludes that the unique errors are not correlated with the regressors.
Variable Significance
P values were also used to select the significant variables from the model by choosing the variables with p values <5% since a 95% confidence interval for the model was used. Both p and t statistics were analysed, with variables with p values<0.05 and t-values>|2.306| considered significant at the 95% confidence level. The t-statistic for the statistically significant variables is shown in parentheses at the respective significance level as shown in the notes for Table 5 above.
From the RE results in Table 5, it is evident that PC 15, PC 13 and PC 1 representing the ratios Capital, Asset Quality and Earnings, which can be represented by the acronym CAE, are the most significant factors which influence bank stability in Zimbabwe. Statistical significance was also established on the end-period CPI as a bank distress causality factor in Zimbabwe.
Resultantly the following equation was used to fit the model used in the bank distress prediction:
Y = 18.49 – 162.58PC15 – 27.97PC13 – 15.81PC1 + 84.43CPI…………….…Equation 5.8
PC 15 is a linear combination of capital and leverage, being proxies to capital in a bank. The component has a huge contribution to bank distress as shown by its beta value of -162.58, which is the largest absolute value of the regressors as shown in Table 4 above. The magnitude of the capital contribution to bank stability is consistent with the proponents of the resource-based view who argue that a well-resourced bank is poised to perform better than less capitalised banks, hence posing a comparative advantage in the market which will result in its capacity to underwrite more business or to absorb incidental business loses.
The PC has a composite negative sign, which is consistent with the a priori expectations of the two ratios embedded in it, being CAR and LVR. The negative sign implies that an increase in PC 15 results in a negative contribution in the calculated Z-score. The more negative the Z score value becomes the less likelihood for the bank to experience distress.
PC 13 – Asset quality
Credit risk is the greatest risk for lending banks. This risk is assessed by analysing the asset quality of a bank’s portfolio. PC 13 is a linear combination of the NPL, NPLA, and IAR ratios which all measure the extent to which a bank is exposed to NPLs.
PC 13 has a negative composite sign, which denotes a negative contribution to the Z score. Its magnitude of 27.973 implies that it exerts less significant weight compared to PC15. From a priori the variables in PC 13 negatively impact on bank stability. This infers that if the asset quality ratio values have got a high absolute positive value (denoting a deteriorating loan book quality), the higher the probability of a bank to experience financial distress, which is consistent with a priori expectations.
PC 1 – Earnings
PC 1 is a combination of the ROE, ROA, and NIM ratios which measure the profitability of a bank. As the bank’s net income relative to its balance sheet size increases the less likely it is to face distress. On the contrary, a distressed bank is bound to generate relatively lower earnings, which reduces its capability to absorb business losses when they occur.
PC 1 has a coefficient 15.81% which signifies the weight of earnings in the prediction model. The overall negative sign of the PC is again consistent with a priori expectations of the embedded income ratios.
Constant term
The t-statistic of the constant term at |0.55| is inconsistent with the earlier stated selection criteria of variable significance. Statistically significant variables are expected to have a t-statistic of greater than |2.306|. The constant term was, however, incorporated into the model to account for the inherent likelihood of distress in any bank which may result from other unobserved factors such as location, bank size, and brand.
Other variables
There was no statistical significance found at the 5% significance level of the relevance of the other variables in the model, such as the management capability and the bank’s liquidity, level in influencing bank distress in Zimbabwe. Conventionally, banks with efficient management and healthier liquidity levels have a greater chance of survival during episodes of financial crisis. The impact on bank performance of these financial ratios may have been subsumed and dominated by the key predictive ratios which displayed statistical significance at the 95% confidence interval.
Control variables
Of the 3 control variables utilised in the model, significant influence on bank stability was established on the end-period CPI at the 5% significance level. This implies that inflation is a crucial macroeconomic factor in influencing bank distress. The distress can manifest itself in the real interest rates which may prevail in the country in the event of rising inflation, thereby causing increased loan defaults.
There is however no statistically significant evidence found in the Nominal Average Lending Rates (NALR) and GDP growth in influencing the viability of banks in Zimbabwe at the 5% significance level. These factors, however, have significance in determining the viability of banks on a broader level. The effects of advancing loans at moderate rates which discourage the moral hazard problem have also been theoretically and empirically proven to influence bank stability around the globe. Studies have also linked improved financial stability of banks in periods of rising GDP output in the economy.
Residuals analysis
Residuals analysis was carried out after fitting the model to check for the normality of the response variables. The results are plotted on the histogram and the Probability to Probability plot (P-P), shown in Figure 3 below:
Figure 3: Residuals Plot
Notes: The residuals as plotted on both the histogram and P-P plot depict normality in the distribution.
Source: Author’s own, 2015.
The residuals exhibit a normal distribution; hence the model is acceptable and fit for purpose. This implies that the sample is derived from a normal distribution.
Distress Prediction
The bank distress prediction process was carried out in a stepwise fashion, following the four stages outlined below:
Observed PD
Annual CAR and LAR ratios for all the banks were examined against the RBZ statutory minimum levels of 12% and 30% respectively. These thresholds were jointly applied, and a bank had to comply with both for it to be deemed stable, otherwise it was deemed distressed. Banks which met the two requirements were deemed stable and had a 0% probability of distress. On the contrary, banks which failed to comply with both thresholds jointly were deemed distressed and were assigned a probability of distress of 1.
Observed Z score
After assigning the probabilities, Z scores were assigned to the 114 bank episodes under investigation. These scores are essential for running the linear regression models which were estimated. Banks with an observed probability of default of 1 were allocated a Z score of 10, whilst the non-distressed banks were allocated a Z score of -10.
Implied Z score
In order to estimate the probability of distress for banks, the implied Z score for the banks had to be calculated using the fitted model in equation 5.8 shown below:
Y = – 18.49 – 162.58PC15 – 27.97PC13 – 15.81PC1 + 84.43CPI
, where Y denotes the Z score
The Z score from the model above is a linear combination of the statistically significant explanatory variables identified from the RE model.
Model PD
The implied Z score above was fed as input in the logit function below to calculate the expected Probability of Distress (PD) from the model.
PD = 1/(1 + exp( – y))…………………………………………………..Equation 5.9,
where y= Z score.
This model used 1 year data on a trailing basis to calculate the probability of distress. Resultantly the model has a predictive forecasting horizon of 12 months.
Model Simulation and testing
The model above was simulated using data from selected banks and periods within the study period. These results were compared against obtainable CAMEL(S) ratings for the banks and the known status of the bank during the time.
Under the CAMELS system, ratings between a scale of ‘1’ (for the best) and ‘5’ (for the worst) were awarded to each of the component factors. As an abridgement of the component ratings, a composite rating was awarded to the bank as it is more reflective of the bank’s current financial position.
In order to maintain uniformity in the research, the study utilised the factors represented in CAMEL to compare results with the model. This left out the sensitivity factor, since it was not part of this investigation due to data availability problems in the market.
The simulation exercise produces very encouraging results with Ecobank, Metbank, Steward Bank, Tetrad and Barclays being classic examples whose empirical behaviour mimicked the model prediction almost consistently over the observation period.
In as much as the model was acceptable in its predictive capacity of distress in the country, it also produced some anomalous results. Banks like Stanbic bank and CBZ had episodes where the model prediction was not consistent with expectations as well as the prevailing RBZ CAMELS rating for the banks. These anomalies could be a result of the fact that the variables used to determine the observed PD narrowly missed the regulatory thresholds despite being in the higher echelons. The irregularities can also be attributed to the endogenous Type II error within the model. The model as shown has an overall R-Square value of 40.55% leaving room for Type II error up to 59.45%.
Robustness Check
The Random Effects model identified the ratios CAE as the most significant predictors of bank distress at the 95% confidence interval. For robustness checking, the regression process was re-estimated using the Population Average (PA) method. Data analysis by the PA produced similar results to the Random Effects (RE) model. It identified the same factors which influence bank distress in Zimbabwe. The notable difference was the significance level of the end-period CPI variable. Under the RE model end-period CPI was significant at the 5% level, whilst the level of significance improved to 1% in the PA model results. The PA results are a positive observation which go a long way to validating the results estimated from the RE model.
Conclusion
The RE model identified the Capital, Asset Quality and Earnings (CAE) ratios with a strong ability to explain bank distress in Zimbabwe. These CAMEL type ratios relate to the bank’s capitalisation, loan book performance, and profitability. The results are consistent with academic theory and other empirical studies conducted in the region. On the macroeconomic control variables, statistical significance at the 5% level was established on the end-period CPI in influencing bank stability, whilst GDP growth and the Nominal Average Lending Rates (NALR) had no statistical significance at that level.
Funding
This research received no specific grant from any funding agency in the public, commercial or not-for-profit sectors.
Notes
- The concept of creation of an SPV by central banks or Governments to house NPLs has been utilised in other countries which experienced credit distress in their banking systems. Typical examples include the Indonesian Bank Restructuring Agency, the Danaharta in Malaysia, the Asset Management Corporation of Nigeria and the Korea Asset Management Company, inter alia.
- Gunsel (2010) established that the ratio of private sector loans on the bank’s portfolio had a direct positive influence on bank stability chances in Cyprus.
References
Adeyeye, P.O., Fajembola, O.D., Olopete, M.O. & Adedeji, D.B. 2012. Predicting Bank Failure in Nigeria using Principal Component Analysis and D-Score Model, Research Journal of Finance and Accounting, 3(8), 159-170.
Alhassan, A.L., Kyereboah-Coleman, A. & Andoh, C. 2014. Asset Quality in a crisis period: An empirical examination of Ghanaian banks, Review of Development Finance, 4, 50-62.
Arabi, K.A.M. 2013. Predicting Bank’s Failure: The Case of Banking Sector in Sudan for the Period of 2002-2009, Journal of Business Studies, 4(3), 160-172.
Athanasoglou, P.P., Brissimis, S.N. & Delis, M.D. 2008. Bank specific, industry-specific and macroeconomic determinants of bank profitability, Journal of international financial markets & money, 18, 121-136.
Baki, M. 2013. How can Bank Reforms Assuage Socioeconomic ordeals in Emerging Economies? Lessons for Egypt from the Turkish Experience, Journal of Developing Areas, 47(2), 37-59.
Bank of Greece. 2008. Working Paper, Exploring the Nexus between banking sector reform and performance: Evidence from newly accentuated EU countries.
Bank of International Settlements. 2001. Marrying the Macro and Micro Prudential Dimensions of Financial Stability, BIS papers, 1.
Barth, J.R., Caprio, G. Jr. & Levine, R. 2001. Banking Systems Around the Globe: Do Regulation and Ownership Affect Performance and Stability? 31-96.
Basel Committee on Bank Supervision. 2014. Basel III leverage ratio framework and disclosure requirements. Bank for International Settlements.
Beck, T., Demirgüç-Kunt, A. & Levine, R. 2004. Bank Concentration & Crisis: First Results, Research Gate Publications. Chicago: University of Chicago Press, 31-96.
Bikker, J.A. & Bos, J.W.B. 2008. Bank Performance: A theoretical and empirical framework for the analysis of profitability, competition and efficiency. New York: Routledge.
Bollard, A., Hunt, C. & Hodgetts, B. 2011. The role of banks in an economy – Improving the Performance of the New Zealand banking system after the global financial crisis, BIS central bankers’ speeches, Tauranga, New Zealand, 1-10.
Brooks, C. 2014. Introductory Econometrics for Finance, Third edition. United Kingdom: Cambridge University Press.
Christopoulous, A.G., Mylonakis, J. & Diktapanidis, P. 2011. Could Lehman Brother’s Collapse be anticipated? An examination using the CAMELS rating system, Journal of International Business Research, 4(2), 11-19.
Claessens, S., Evanoff, D.D., Kaufman, G.G. & Kodres, L.E. 2011. Macro prudential Regulatory Policies: The New Road to Financial Stability, World Scientific Publishing Company.
Corsetti, G., Pesenti, P. & Roubiniet, N. 2001. Fundamental Determinants of the Asian Crisis, The role of Financial Fragility and External Imbalance, University of Chicago Press, National Bureau of Economic Research, 11-41.
Dabos, M. & Escudero, W.S. 2004. Explaining and Predicting Bank Failure Using Duration Models: The Case of Argentina after the Mexican Crisis, Revista de Análisis Económico, 19(1), 31-49.
Dallas Fed. 2013. How bad was it? The costs and Consequences of the 2007-09 Financial Crisis, Staff Papers, 20.
De Larosière Group. 2009. The High-Level Group on Financial Supervision in the EU, Chaired by Jacques de Larosière.
Eernisse, A.C. 2012. Banking on Cooperation: The role of the G-20 in improving the international financial architecture, Duke Journal of comparative & International law, 22(239), 239-266.
Federal Reserve Bank of Dallas. 2011. Hyperinflation in Zimbabwe, Globalisation and Monetary policy Institute, 2011 Annual Report.
Fowowe, B. 2013. Financial Liberalization in Sub-Saharan Africa: What do we Know, Journal of Economic Surveys, 27(1), 1-37.
Gunsel, N. 2010. Determinants of the timing of bank failure in North Cyprus, Journal of risk finance, 11(1), 89-106.
Gupta, R. 2014. An analysis of Indian public sector banks using Camels approach, Journal of Business Management, 16(1), 94-102.
Haq, M. & Heaney, R. 2012. Factors Determining European bank risk, Journal of International Financial Markets, Institutions & Money, 22, 696-718.
Holland, J. 2010. Banks, Knowledge and Crisis: A Case of knowledge and Learning Failure, Journal of Financial Regulation & Compliance, 18(2), 87-105.
Hsu, B.F.C., Arner, D. & Pretorius, F. 2007. Beyond the Basel Accord: Should the Capital Adequacy Ratio Take Account of the Real Estate Environment in Hong Kong Sar. Banking Law Journal, 124(4), 297-308.
Huang, D.T., Chang, B. & Liu Z.C. 2010. Bank failure prediction models: for the developing and developed countries, 46, 553–558.
Hutchison, M.M. 2002. European Banking Distress and EMU: Institutional and Macroeconomic Risks, Journal of Economics, 104(3), 365–389.
IFC. 2009. Proceedings of the IFC Conference on “Measuring financial innovation and its impact”, Bulletin No 31.
IMF. 1997. Liquid Asset ratios and Financial Sector Reform, IMF Working Paper, WP/97/144.
IMF. 2006. Working Paper, How do Central Banks write on Financial Stability, Number 06/163.
IMF. 2014. Global Financial Stability Report: Risk Taking, Liquidity and Shadow banking -Curbing Excess While Promoting Growth.
Karminsky, A. & Kostrov, A. 2014. Comparison of Bank Stability Factors in CIS Countries, Procedia Computer Science, 31, 766-772.
Keller, G. 2012. Managerial Statistics, 9th Edition, International Edition. USA: South Western Cengage Learning.
Koop, G. 2009. Analysis of Economic Data. Amazon Warehouse.
Krueger, R. 2002. International Standards for Impairment and Provisions and their Implications for financial Soundness Indicators (FSIs). IMF Working Paper, 1-25.
Kurmann, P. & Bessler. W. 2014. Bank Risk factors and changing risk exposures: Capital market evidence before and during the financial crisis, Journal of financial stability, 13, 151-166.
Louzis, D.P., Vouldis, A. T. & Metaxas, V.L. 2011. Macroeconomic and bank specific determinants of nonperforming loans in Greece: A comparative study of mortgage, business and consumer loan portfolios, Journal of Banking and finance, 36, 1012-1027.
McArthur, J.W., Schmidt-Traub, G., Kruk, M., Bahadur, C., Faye, M. & McCord, G. 2004. Ending Africa’s Poverty Trap, Brookings Papers on Economic Activity, 1, 117-216.
McCune, J. 2008. Tier 1 ratios: Stable so far, ABA Banking Journal, SNL Financial.
Maghyereh, A.I. & Awartani, B. 2013. Bank distress prediction: Empirical evidence from the gulf cooperation council countries. Research in International Business and Finance, 30, 126-147.
Mamiza, H. & Heaney, R. 2012. Factors determining European bank risk, Journal of International Financial Markets, 22(1), 696-718.
Mannasso, K. & Mayes, D.G. 2009. Explaining Bank Distress in Eastern European Transition Economies, Journal of Banking & Finance, 33, 244-253.
Moyo, J., Nandwa, B., Oduor, J. & Simpasa, A. 2014. Financial Sector Reforms, Competition and Banking System Stability in Sub-Saharan Africa, Paper Presented at a conference on Macroeconomic Challenges Facing Low-Income Countries, hosted by IMF & DFID. Washington DC.
Moyo, T. 1999. Financial Sector Liberalization and the Poor: A Critical Appraisal. . Paper presented For the SAPRI – Zimbabwe Initiative Poverty Reduction Forum, Harare, Zimbabwe.
Nuxoll, D.A. 2003. The Contribution of Economic Data to Bank-Failure Models, FDIC Working Paper, 2003-03.
Petitjean, M. 2013. Bank Failures and Regulation, Journal of Financial Regulation and Compliance, 21(1), 16-38.
Quagliariello, M. 2008. Does macro economy affect bank stability? A review of the empirical, Journal of Banking Regulation, 9(2), 102–115.
Reserve Bank of Zimbabwe. 2014. Financial Stability Report. June 2014.
Reserve Bank of Zimbabwe. 2015. Monetary Policy Statement. January 2015.
Tefula, M. & Murinde, V. 2002. Identifying Problem Banks in Sub-Saharan Africa: Africa Problem Banks, the African Finance Journal, 4(2).
Torres-Reyna, O. 2007. Panel Data Analysis: Fixed and Random Effects using Stata, Data and Statistical Services. United States: Princeton University.
Whitaker, R.B. 1999. The early stages of financial distress, Journal of Economics and Finance, 23(2), 123-132.
Wingard, H.C., Von Well, R., Pretorius, D., Ferreira, P.H., Badenhorst, V.M. & van der Merwe, D. 2013. GAAP HANDBOOK: Financial Accounting and Reporting Practice, Volume 1, Lexis Nexis.
World Bank. 2008. Bank Competition and Financial Stability: Friends or Foes? Policy Research Working Paper 4656.
World Bank. 2010. Corporate Vulnerability and Bank Stability: Evidence from Jordan, Policy Research Working Paper 5502.
Zaki, E., Bah, R. & Rao, A. 2011. Assessing probabilities of financial distress of banks in UAE, International Journal of Managerial Finance, 7(3), 304-320.
Zimbabwe National Statistics Agency. 2015. Quarterly Digest of Statistics, 1st Quarter, April.